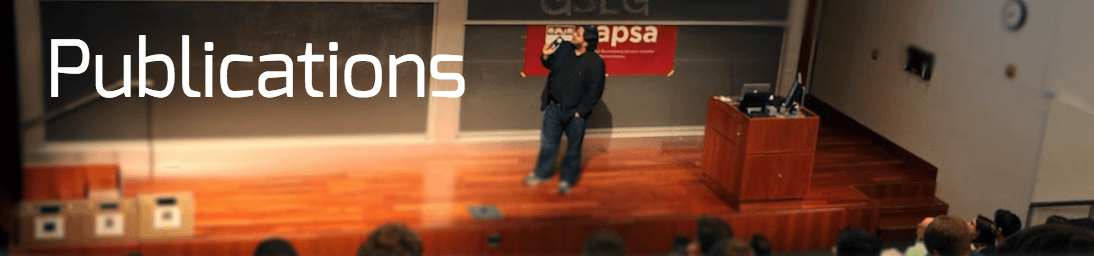
Dissertation
Madhur Behl (2015) Ph.D. Thesis Electrical & Systems Engineering "Data-driven modeling, control and tools for cyber-physical energy systems", Univeristy of Pennsylvania. Available from Dissertations & Theses @ University of Pennsylvania; ProQuest Dissertations &Theses Global. (1761166227) pp. 138. | |||||
BibTeX:
@misc{ author={Behl,Madhur}, year={2015}, title={Data-driven modeling, control and tools for cyber-physical energy systems}, journal={ProQuest Dissertations and Theses}, pages={138}, note={Copyright - Copyright ProQuest, UMI Dissertations Publishing 2015; Last updated - 2016-02-01}, keywords={Applied sciences; Control; Cyber-physical systems; Data-driven; Demand response; Energy; Regression tree; Electrical engineering; Computer science; 0984:Computer science; 0791:Energy; 0544:Electrical engineering}, isbn={9781339429656}, language={English}, url={http://search.proquest.com/docview/1761166227?accountid=14707}, } } |
|||||
Abstract: Energy systems are experiencing a gradual but substantial change in moving away from being non-interactive and manually-controlled systems to utilizing tight integration of both cyber (computation, communications, and control) and physical representations guided by first principles based models, at all scales and levels. Furthermore, peak power reduction programs like demand response (DR) are becoming increasingly important as the volatility on the grid continues to increase due to regulation, integration of renewables and extreme weather conditions. In order to shield themselves from the risk of price volatility, end-user electricity consumers must monitor electricity prices and be flexible in the ways they choose to use electricity. This requires the use of control-oriented predictive models of an energy system’s dynamics and energy consumption. Such models are needed for understanding and improving the overall energy efficiency and operating costs. However, learning dynamical models using grey/white box approaches is very cost and time prohibitive since it often requires significant financial investments in retrofitting the system with several sensors and hiring domain experts for building the model. We present the use of data-driven methods for making model capture easy and efficient for cyber-physical energy systems. We develop Model-IQ, a methodology for analysis of uncertainty propagation for building inverse modeling and controls. Given a grey-box model structure and real input data from a temporary set of sensors, Model-IQ evaluates the effect of the uncertainty propagation from sensor data to model accuracy and to closed-loop control performance. We also developed a statistical method to quantify the bias in the sensor measurement and to determine near optimal sensor placement and density for accurate data collection for model training and control. Using a real building test-bed, we show how performing an uncertainty analysis can reveal trends about inverse model accuracy and control performance, which can be used to make informed decisions about sensor requirements and data accuracy. We also present DR-Advisor, a data-driven demand response recommender system for the building's facilities manager which provides suitable control actions to meet the desired load curtailment while maintaining operations and maximizing the economic reward. We develop a model based control with regression trees algorithm (mbCRT), which allows us to perform closed-loop control for DR strategy synthesis for large commercial buildings. Our data-driven control synthesis algorithm outperforms rule-based demand response methods for a large DoE commercial reference building and leads to a significant amount of load curtailment (of 380kW) and over $45,000 in savings which is 37.9% of the summer energy bill for the building. The performance of DR-Advisor is also evaluated for 8 buildings on Penn's campus; where it achieves 92.8% to 98.9% prediction accuracy. We also compare DR-Advisor with other data driven methods and rank 2nd on ASHRAE's benchmarking data-set for energy prediction. |
Book Chapters
Madhur Behl and Rahul Mangharam (2017), "Chapter 9: Data-Driven Modeling, Control, and Tools for Smart Cities", Pg 243-272, Book - Smart Cities: Foundations and Principles, John Wiley & Sons Inc, ISBN: 978-1-119-22639-0, June 2017
[URL] |
Journal Papers
[13] Aron Harder, Amar Kulkarni, and Madhur Behl."HALO: Fault-Tolerant Safety Architecture for High-Speed Autonomous Racing", ACM Transactions on Cyber-Physical SYstems. 2022. [Under Review] | |||||
BibTeX:
|
|||||
Abstract: High-speed autonomous racing has become an active area of research in recent years. Full-scale autonomous racing competitions such as RoboRace and the Indy Autonomous Challenge have given researchers an opportunity to develop the software stack for high-performance race vehicles which aim to operate autonomously on the edge of the vehicle's limits. These autonomous race cars can race at speeds greater than 150mph, while overtaking another autonomous race car. In order for the autonomous race car to achieve an acceptable level of safety, the software must actively monitor for erroneous operating conditions, as well as faults and errors during its high-speed operation. Achieving safety means ensuring the absence of unreasonable risks due to hazards caused by a malfunction in any of the software's sub-systems and components. While there exist open-source self-driving stacks such as Autoware and Apollo, there is a lack of a comprehensive examination of the safety aspects of autonomous driving at a programming and functional level. This paper presents the first holistic overview of a safety architecture called HALO which has been implemented on a full-scale fully autonomous racing vehicle as part of the Indy Autonomous Challenge. We present a failure mode and criticality analysis for the software stack that could occur in the perception, planning, control, and communication modules. We then provide safety archetypes and runtime monitoring methods to mitigate these faults. We demonstrate the effectiveness of HALO on data gathered from real-world runs of our autonomous race car. | |||||
[12] Varundev Suresh Babu and Madhur Behl."ARGOS: an Automaton Referencing Guided Overtake System for Head-to-Head Autonomous Racing", IEEE Transactions on Robotics (T-RO). 2022. [Under Review] | |||||
BibTeX:
@conference{babu22, title={ARGOS: an Automaton Referencing Guided Overtake System for Head-to-Head Autonomous Racing}, author={\underline{Varundev Sukhil} and Madhur Behl}, booktitle={IEEE Transactions on Robotics (T-RO)}, year={2022}, Note={[Under Review]} } |
|||||
Abstract: | |||||
[11] Betz, Johannes and Zheng, Hongrui and Liniger, Alexander and Rosolia, Ugo and Karle, Phillip and Behl, Madhur and Krovi, Venkat and Mangharam, Rahul."Autonomous Vehicles on the Edge: A Survey on Autonomous Vehicle Racing", IEEE Open Journal of Intelligent Transportation Systems. 2022. | |||||
BibTeX:
@article{johannes, title={Autonomous Vehicles on the Edge: A Survey on Autonomous Vehicle Racing}, author={Betz, Johannes and Zheng, Hongrui and Liniger, Alexander and Rosolia, Ugo and Karle, Phillip and Behl, Madhur and Krovi, Venkat and Mangharam, Rahul}, journal={IEEE Open Journal of Intelligent Transportation Systems}, year={2022}, note={[Impact Factor: 4.277]} } |
|||||
Abstract: The rising popularity of self-driving cars has led to the emergence of a new research field in the recent years: Autonomous racing. Researchers are developing software and hardware for high performance race vehicles which aim to operate autonomously on the edge of the vehicles limits: High speeds, high accelerations, low reaction times, highly uncertain, dynamic and adversarial environments. This paper represents the first holistic survey that covers the research in the field of autonomous racing. We focus on the field of autonomous racecars only and display the algorithms, methods and approaches that are used in the fields of perception, planning and control as well as end-to-end learning. Further, with an increasing number of autonomous racing competitions, researchers now have access to a range of high performance platforms to test and evaluate their autonomy algorithms. This survey presents a comprehensive overview of the current autonomous racing platforms emphasizing both the software-hardware co-evolution to the current stage. Finally, based on additional discussion with leading researchers in the field we conclude with a summary of open research challenges that will guide future researchers in this field. | |||||
[10] Varundev Suresh Babu and Madhur Behl."Threading the Needle—Overtaking Framework for Multi-agent Autonomous Racing", SAE International Journal of Connected and Automated Vehicles, 5(1). 10.4271/12-05-01-0004. Jan 2022 | |||||
BibTeX:
@article{12-05-01-0004, author={\underline{Suresh Babu, Varundev} and Behl, Madhur}, title={Threading the Needle—Overtaking Framework for Multi-agent Autonomous Racing}, journal={SAE International Journal of Connected and Automated Vehicles}, volume={5}, number={1}, month={Jan}, year={2022}, doi={https://doi.org/10.4271/12-05-01-0004}, url={https://doi.org/10.4271/12-05-01-0004}, issn={2574-0741} } |
|||||
Abstract: Multi-agent autonomous racing still remains a largely unsolved research challenge. The high-speed and close proximity situations that arise in multi-agent autonomous racing present an ideal condition to design algorithms which trade off aggressive overtaking maneuvers and minimize the risk of collision with the opponent. In this article we study a two-vehicle autonomous racing setup and present AutoPass—a novel framework for overtaking in a multi-agent setting. AutoPass uses the structure of an automaton to break down the complex task of overtaking into sub-maneuvers that balance overtaking likelihood and risk with safety of the ego vehicle. We present real-world implementation of 1/10-scale autonomous racing cars to demonstrate the effectiveness of AutoPass for the overtaking task. Our results indicate that the overtake success ratio for the AutoPass framework is 0.395, or 23 times more likely, compared to a purely reactive system at 0.017, while traditional Robot Operating System (ROS)-based path planners (depending on the navigation plugin used) are placed between 0.115 and 0.286. | |||||
[9] Praharaj, Shraddha & Chen, T. Donna & Zahura, Faria & Behl, Madhur & Goodall, Jonathan."Estimating impacts of recurring flooding on roadway networks: a Norfolk, Virginia case study", 2021 Journal of Natural Hazards, 1-25. 10.1007/s11069-020-04427-5. | |||||
BibTeX:
@article{shraddhahazard21, author = {Praharaj, Shraddha and Chen, T. Donna and Zahura, Faria and Behl, Madhur and Goodall, Jonathan}, year = {2021}, month = {01}, pages = {1-25}, title = {Estimating impacts of recurring flooding on roadway networks: a Norfolk, Virginia case study}, journal = {Natural Hazards}, doi = {10.1007/s11069-020-04427-5} } |
|||||
Abstract: Climate change and sea level rise have increased the frequency and severity of flooding events in coastal communities. This study quantifies transportation impacts of recurring flooding using crowdsourced traffic and flood incident data. Agency-provided continuous count station traffic volume data at 12 locations is supplemented by crowd-sourced traffic data from location-based apps in Norfolk, Virginia, to assess the impacts of recurrent flooding on traffic flow. A random forest data predictive model utilizing roadway features, traffic flow characteristics, and hydrological data as inputs scales the spatial extent of traffic volume data from 12 to 7736 roadway segments. Modeling results suggest that between January 2017 and August 2018, City of Norfolk reported flood events reduced 24 h citywide vehicle-hours of travel (VHT) by 3%, on average. To examine the temporal and spatial variation of impacts, crowdsourced flood incident reports collected by navigation app Waze between August 2017 and August 2018 were also analyzed. Modeling results at the local scale show that on weekday afternoon and evening periods, flood-impacted areas experience a statistically significant 7% reduction in VHT and 12% reduction in vehicle-miles traveled, on average. These impacts vary across roadway types, with substantial decline in traffic volumes on freeways, while principal arterials experience increased traffic volumes during flood periods. Results suggest that analyzing recurring flooding at the local scale is more prudent as the impact is temporally and spatially heterogeneous. Furthermore, countermeasures to mitigate impacts require a dynamic strategy that can adapt to conditions across various time periods and at specific locations. | |||||
[8] Bowes, Benjamin D and Tavakoli, Arash and Wang, Cheng and Heydarian, Arsalan and Behl, Madhur and Beling, Peter A and Goodall, Jonathan L"Flood mitigation in coastal urban catchments using real-time stormwater infrastructure control and reinforcement learning", 2020 Journal of Hydroinformatics | |||||
BibTeX:
@article{bowes2020flood, title={Flood mitigation in coastal urban catchments using real-time stormwater infrastructure control and reinforcement learning}, author={Bowes, Benjamin D and Tavakoli, Arash and Wang, Cheng and Heydarian, Arsalan and Behl, Madhur and Beling, Peter A and Goodall, Jonathan L}, journal={Journal of Hydroinformatics}, year={2020} } |
|||||
Abstract: Flooding in coastal cities is increasing due to climate change and sea-level rise, stressing the traditional stormwater systems these communities rely on. Automated real-time control (RTC) of these systems can improve performance, and creating control policies for smart stormwater systems is an active area of study. This research explores reinforcement learning (RL) to create control policies to mitigate flood risk. RL is trained using a model of hypothetical urban catchments with a tidal boundary and two retention ponds with controllable valves. RL's performance is compared to the passive system, a model predictive control (MPC) strategy, and a rule-based control strategy (RBC). RL learns to proactively manage pond levels using current and forecast conditions and reduced flooding by 32% over the passive system. Compared to the MPC approach using a physics-based model and genetic algorithm, RL achieved nearly the same flood reduction, just 3% less than MPC, with a significant 88× speedup in runtime. Compared to RBC, RL was able to quickly learn similar control strategies and reduced flooding by an additional 19%. This research demonstrates that RL can effectively control a simple system and offers a computationally efficient method that could scale to RTC of more complex stormwater systems. | |||||
[7] Zahura, Faria T and Goodall, Jonathan L and Sadler, Jeffrey M and Shen, Yawen and Morsy, Mohamed M and Behl, Madhur, "Training machine learning surrogate models from a high-fidelity physics-based model: Application for real-time street-scale flood prediction in an urban coastal community", 2020 Water Resources Research, Wiley Online Library, Vol. 56(10), pg. e2019WR027038 | |||||
BibTeX:
@article{zahura2020training, title={Training machine learning surrogate models from a high-fidelity physics-based model: Application for real-time street-scale flood prediction in an urban coastal community}, author={Zahura, Faria T and Goodall, Jonathan L and Sadler, Jeffrey M and Shen, Yawen and Morsy, Mohamed M and Behl, Madhur}, journal={Water Resources Research}, volume={56}, number={10}, pages={e2019WR027038}, year={2020}, publisher={Wiley Online Library} } |
|||||
Abstract: Mitigating the adverse impacts caused by increasing flood risks in urban coastal communities requires effective flood prediction for prompt action. Typically, physics‐based 1‐D pipe/2‐D overland flow models are used to simulate urban pluvial flooding. Because these models require significant computational resources and have long run times, they are often unsuitable for real‐time flood prediction at a street scale. This study explores the potential of a machine learning method, Random Forest (RF), to serve as a surrogate model for urban flood predictions. The surrogate model was trained to relate topographic and environmental features to hourly water depths simulated by a high‐resolution 1‐D/2‐D physics‐based model at 16,914 road segments in the coastal city of Norfolk, Virginia, USA. Two training scenarios for the RF model were explored: (i) training on only the most flood‐prone street segments in the study area and (ii) training on all 16,914 street segments in the study area. The RF model yielded high predictive skill, especially for the scenario when the model was trained on only the most flood‐prone streets. The results also showed that the surrogate model reduced the computational run time of the physics‐based model by a factor of 3,000, making real‐time decision support more feasible compared to using the full physics‐based model. We concluded that machine learning surrogate models strategically trained on high‐resolution and high‐fidelity physics‐based models have the potential to significantly advance the ability to support decision making in real‐time flood management within urban communities. | |||||
[6] Gina L O'Neil, Jonathan L Goodall, Madhur Behl, Linnea Saby, "Deep learning Using Physically-Informed Input Data for Wetland Identification", 2020 Environmental Modelling & Software, Vol. 126, pg. 104665 | |||||
BibTeX:
@article{o2020deep, title={Deep learning Using Physically-Informed Input Data for Wetland Identification}, author={O'Neil, Gina L and Goodall, Jonathan L and Behl, Madhur and Saby, Linnea}, journal={Environmental Modelling \& Software}, volume={126}, pages={104665}, year={2020}, publisher={Elsevier} } |
|||||
Abstract: Automated and accurate wetland identification algorithms are increasingly important for wetland conservation and environmental planning. Deep learning for wetland identification is an emerging field that shows promise for advancing these efforts. Deep learning is unique to traditional machine learning techniques for its ability to consider the spatial context of object characteristics within a landscape scene. However, applying deep learning typically requires very large datasets for training the algorithms, which limits their application for many environmental applications including wetland identification. Using four study sites across Virginia with field delineated wetlands, we provide insight into the potential for deep learning for wetland detection from limited, but typical, wetland delineation training data. Our proposed workflow performs a wetland semantic segmentation using DeepNets, a deep learning architecture for remote sensing data, and an input dataset consisting of high-resolution topographic indices and the Normalized Difference Vegetation Index. Results show that models trained and evaluated for a single site were able to achieve high accuracy (up to 91% recall and 56% precision) and similar accuracy can be obtained for models trained across multiple sites (up to 91% recall and 57% precision). Through this analysis we found that, across all sites, input data configurations taking advantage of hydrologic properties derived from elevation data consistently outperformed models using the elevation data directly, showing the benefit of physically-informed inputs in deep learning training for wetland identification. By refining the wetland identification workflow presented in this paper and collecting additional training data across landscapes, there is potential for deep learning algorithms to support a range wetland conservation efforts. | |||||
[5] Jeffrey M Sadler, Jonathan L Goodall, Madhur Behl, Benjamin D Bowes, Mohamed M Morsy, "Exploring real-time control of stormwater systems for mitigating flood risk due to sea level rise", 2020 Journal of Hydrology, Vol. 583, pg. 124571 | |||||
BibTeX:
@article{sadler2020exploring, title={Exploring real-time control of stormwater systems for mitigating flood risk due to sea level rise}, author={Sadler, Jeffrey M and Goodall, Jonathan L and Behl, Madhur and Bowes, Benjamin D and Morsy, Mohamed M}, journal={Journal of Hydrology}, volume={583}, pages={124571}, year={2020}, publisher={Elsevier} } |
|||||
Abstract: Low-lying, low-relief coastal cities have seen increased flooding due to climate change. In these cities, stormwater pipe outlets can be inundated by coastal waters at high tide or from storm surge, making drainage impossible. The objective of this research is to assess the utility of model predictive control (MPC) of stormwater actuators to reduce flooding in a coastal urban setting made worse by sea level rise. The stormwater system and the control scenarios are simulated using the United States Environmental Protection Agency Storm Water Management Model (SWMM5) coupled with a Python library, swmm_mpc. The study area is Norfolk, Virginia, USA, a city which is particularly vulnerable to coastal flooding. A simulated 2-year 12-h design storm and sea level rise scenarios up to 3.5 ft are applied to the model for three control scenarios: 1) a passive system, 2) a passive system with a tide gate (backflow preventer), and 3) a tide gate and three actuators (a pump, a valve, and an inflatable dam) controlled through MPC. Flooding in the passive system reached a tipping point and increased dramatically after a sea level rise of 1.6 ft. The addition of a tide gate greatly mitigated this increase in flooding. MPC further reduced overall flooding with an average effective percent reduction of 32%. The rate of the increase in flooding was significantly smaller with MPC than without. MPC also reduced maximum node flood volume by an average of 52% for sea level increases of 2.0 ft and above. In addition to the installation of a tide gate, our results suggest that the use of actuators controlled by MPC could significantly reduce overall flood volumes and reduce flood severity at individual nodes in coastal cities. | |||||
[4] Jeffrey M Sadler, Jonathan L Goodall, Madhur Behl, Mohamed M Morsy, Teresa B Culver, Benjamin D Bowes, "Leveraging open source software and parallel computing for model predictive control of urban drainage systems using EPA-SWMM5", 2019 Journal of Environmental Modelling & Software, Vol. 120, pg. 104484 | |||||
BibTeX:
@article{sadler2019leveraging, title={Leveraging open source software and parallel computing for model predictive control of urban drainage systems using EPA-SWMM5}, author={Sadler, Jeffrey M and Goodall, Jonathan L and Behl, Madhur and Morsy, Mohamed M and Culver, Teresa B and Bowes, Benjamin D}, journal={Environmental Modelling \& Software}, volume={120}, pages={104484}, year={2019}, publisher={Elsevier} } |
|||||
Abstract: Active stormwater control will play an increasingly important role in mitigating urban flooding, which is becoming more common with climate change and sea level rise. In this paper we describe and demonstrate swmm_mpc, software developed for simulating model predictive control (MPC) for urban drainage systems using open source software (Python and the EPA Stormwater Management Model version 5 (SWMM5)). Swmm_mpc uses an evolutionary algorithm as an optimizer and supports parallel processing. In the demonstration case for a hypothetical, tidally-influenced urban drainage system, the swmm_mpc control policies for two storage units achieved its objectives of 1) practically eliminating flooding and 2) maintaining the water level at the storage units close to a target level. Although the current swmm_mpc workflow was feasible for a simple model using a desktop PC, a high-performance computer or cloud-based computer with more computational cores would likely be needed for most real-world models. | |||||
[3] Benjamin D Bowes, Jeffrey M Sadler, Mohamed M Morsy, Madhur Behl, Jonathan L Goodall, "Forecasting groundwater table in a flood prone coastal city with long short-term memory and recurrent neural networks", 2019 Journal of Water, Vol. 11, Issue 5, pg. 1098 [2021 Journal Best Paper Award] | |||||
BibTeX:
@article{bowes2019forecasting, title={Forecasting groundwater table in a flood prone coastal city with long short-term memory and recurrent neural networks}, author={Bowes, Benjamin D and Sadler, Jeffrey M and Morsy, Mohamed M and Behl, Madhur and Goodall, Jonathan L}, journal={Water}, volume={11}, number={5}, pages={1098}, year={2019}, publisher={Multidisciplinary Digital Publishing Institute} } |
|||||
Abstract: Many coastal cities are facing frequent flooding from storm events that are made worse by sea level rise and climate change. The groundwater table level in these low relief coastal cities is an important, but often overlooked, factor in the recurrent flooding these locations face. Infiltration of stormwater and water intrusion due to tidal forcing can cause already shallow groundwater tables to quickly rise toward the land surface. This decreases available storage which increases runoff, stormwater system loads, and flooding. Groundwater table forecasts, which could help inform the modeling and management of coastal flooding, are generally unavailable. This study explores two machine learning models, Long Short-term Memory (LSTM) networks and Recurrent Neural Networks (RNN), to model and forecast groundwater table response to storm events in the flood prone coastal city of Norfolk, Virginia. To determine the effect of training data type on model accuracy, two types of datasets (i) the continuous time series and (ii) a dataset of only storm events, created from observed groundwater table, rainfall, and sea level data from 2010–2018 are used to train and test the models. Additionally, a real-time groundwater table forecasting scenario was carried out to compare the models’ abilities to predict groundwater table levels given forecast rainfall and sea level as input data. When modeling the groundwater table with observed data, LSTM networks were found to have more predictive skill than RNNs (root mean squared error (RMSE) of 0.09 m versus 0.14 m, respectively). The real-time forecast scenario showed that models trained only on storm event data outperformed models trained on the continuous time series data (RMSE of 0.07 m versus 0.66 m, respectively) and that LSTM outperformed RNN models. Because models trained with the continuous time series data had much higher RMSE values, they were not suitable for predicting the groundwater table in the real-time scenario when using forecast input data. These results demonstrate the first use of LSTM networks to create hourly forecasts of groundwater table in a coastal city and show they are well suited for creating operational forecasts in real-time. As groundwater table levels increase due to sea level rise, forecasts of groundwater table will become an increasingly valuable part of coastal flood modeling and management. | |||||
[2] Achin Jain, Rahul Mangharam, and Madhur Behl (2017), "Data-driven model predictive control with regression trees—An application to building energy management", 2018 ACM Transactions on Cyber-Physical Systems | |||||
BibTeX:
@article{jain2018data, title={Data-driven model predictive control with regression trees—An application to building energy management}, author={Jain, Achin and Smarra, Francesco and Behl, Madhur and Mangharam, Rahul}, journal={ACM Transactions on Cyber-Physical Systems}, volume={2}, number={1}, pages={1--21}, year={2018}, publisher={ACM New York, NY, USA} } |
|||||
Abstract: Model Predictive Control (MPC) plays an important role in optimizing operations of complex cyber-physical systems because of its ability to forecast system’s behavior and act under system level constraints. However, MPC requires reasonably accurate underlying models of the system. In many applications, such as building control for energy management, Demand Response, or peak power reduction, obtaining a high-fidelity physics-based model is cost and time prohibitive, thus limiting the widespread adoption of MPC. To this end, we propose a data-driven control algorithm for MPC that relies only on the historical data. We use multioutput regression trees to represent the system’s dynamics over multiple future time steps and formulate a finite receding horizon control problem that can be solved in real-time in closed-loop with the physical plant. We apply this algorithm to peak power reduction in buildings to optimally trade-off peak power reduction against thermal comfort without having to learn white/grey box models of the systems dynamics. | |||||
[1] Madhur Behl and Francesco Smarra and Rahul Mangharam (2016), "A Data-Driven Demand Response Recommender System", Journal of Applied Energy. | |||||
BibTeX:
@article{BehlMangharam2015, author = {Madhur Behl and Francesco Smarra and Rahul Mangharam}, title = {A Data-Driven Demand Response Recommender System}, journal = {Journal of Applied Energy}, year = {2016}, url = {http://www.sciencedirect.com/science/article/pii/S030626191630246X} } |
|||||
Abstract: Demand response (DR) is becoming increasingly important as the volatility on the grid continues to increase. Current DR ap- proaches are predominantly completely manual and rule-based or involve deriving first principles based models which are ex- tremely cost and time prohibitive to build. We consider the problem of data-driven end-user DR for large buildings which involves predicting the demand response baseline, evaluating fixed rule based DR strategies and synthesizing DR control actions. The challenge is in evaluating and taking control decisions at fast time scales in order to curtail the power consumption of the building, in return for a financial reward. We provide a model based control with regression trees algorithm (mbCRT), which allows us to perform closed-loop control for DR strategy synthesis for large commercial buildings. Our data-driven control synthesis algorithm outperforms rule-based DR by 17% for a large DoE commercial reference building and leads to a curtailment of 380kW and over $45, 000 in savings. Our methods have been integrated into an open source tool called DR-Advisor, which acts as a recommender system for the building’s facilities manager and provides suitable control actions to meet the desired load curtailment while maintaining operations and maximizing the economic reward. DR-Advisor achieves 92.8% to 98.9% prediction accuracy for 8 buildings on Penn’s campus. We compare DR-Advisor with other data driven methods and rank 2nd on ASHRAE’s benchmarking data-set for energy prediction |
[33] Trent Weiss and Madhur Behl, " This is the Way: Differential Bayesian Filtering for Agile Trajectory Synthesis", 2022 IEEE/RSJ International Conference on Intelligent Robots and Systems (IROS) - RA-L Contributed Paper. | |||||
BibTeX:
@conference{trent2021iros, title={This is the Way: Differential Bayesian Filtering for Agile Trajectory Synthesis}, author={\underline{Trent Weiss} and Madhur Behl}, booktitle={2022 IEEE/RSJ International Conference on Intelligent Robots and Systems (IROS)}, year={2022} } } |
|||||
Abstract: One of the main challenges in autonomous racing is to design algorithms for motion planning at high speed, and across complex racing courses. End-to-end trajectory synthesis has been previously proposed where the trajectory for the ego vehicle is computed based on camera images from the racecar. This is done in a supervised learning setting using behavioral cloning techniques. In this paper, we address the limitations of behavioral cloning methods for trajectory synthesis by introducing Differential Bayesian Filtering (DBF), which uses probabilistic B\'ezier curves as a basis for inferring optimal autonomous racing trajectories based on Bayesian inference. We introduce a trajectory sampling mechanism and combine it with a filtering process which is able to push the car to its physical driving limits. The performance of DBF is evaluated on the DeepRacing Formula One simulation environment and compared with several other trajectory synthesis approaches as well as human driving performance. DBF achieves the fastest lap time, and the fastest speed, by pushing the racecar closer to its limits of control while always remaining inside track bounds. | |||||
[32] Jingyun Ning, Benjamin Bowes, Jonathan Goodall and Madhur Behl, " Data-Driven Model Predictive Control For Real-Time Stormwater Management", American Control Conference (ACC) 2022 | |||||
BibTeX:
@conference{jingyunacc, title={Data-Driven Model Predictive Control For Real-Time Stormwater Management}, author={\underline{Jingyun Ning} and Benjamin Bowes and Jonathan Goodall and Madhur Behl}, booktitle={American Control Conference (ACC)}, year={2022}, Note={8 Pages, [Accepted - To Appear][Acceptance Rate: 56\%]} } |
|||||
Abstract: Low-lying coastal cities across the world are increasingly seeing flooding due to climate change and accompanying sea-level rise. Many such cities rely on old and passive stormwater infrastructure which cannot cope up with the increasing flood risk. One potential solution for addressing coastal flooding is implementing active control strategies in the stormwater systems. Existing stormwater control mostly relies on rule-based strategies, which are not sufficient to manage the increasing flood risk. Model predictive control (MPC) for stormwater management has received attention for this problem. However, building physics-based models for MPC in stormwater management is very cost and time prohibitive. In this paper, we propose a data-driven approach, which utilizes unstructured state-space models for system identification and predictive control implementation. We demonstrate our results using two real stormwater network configurations, one from the Norfolk, VA region and another model of Ann Arbor region, MI, respectively using the SWMM simulator. Our results indicate that MPC outperforms rule-based strategies by up to $60\%$ for the Norfolk system and up to $90\%$ for the Ann Arbor system in flood management. | |||||
[31] Aron Harder, Jaspreet Ranjit and Madhur Behl, " Scenario2Vector: scenario description language based embeddings for traffic situations", ICCPS '21: Proceedings of the ACM/IEEE 12th International Conference on Cyber-Physical Systems, Pages 167–176 | |||||
BibTeX:
@inproceedings{10.1145/3450267.3450544, author = {Harder, Aron and Ranjit, Jaspreet and Behl, Madhur}, title = {Scenario2Vector: Scenario Description Language Based Embeddings for Traffic Situations}, year = {2021}, isbn = {9781450383530}, publisher = {Association for Computing Machinery}, address = {New York, NY, USA}, url = {https://doi.org/10.1145/3450267.3450544}, doi = {10.1145/3450267.3450544}, abstract = {A popular metric for measuring progress in autonomous driving has been the "miles per intervention". This is nowhere near a sufficient metric and it does not allow for a fair comparison between the capabilities of two autonomous vehicles (AVs). In this paper we propose Scenario2Vector - a Scenario Description Language (SDL) based embedding for traffic situations that allows us to automatically search for similar traffic situations from large AV data-sets. Our SDL embedding distills a traffic situation experienced by an AV into its canonical components - actors, actions, and the traffic scene. We can then use this embedding to evaluate similarity of different traffic situations in vector space. We have also created a first of its kind, Traffic Scenario Similarity (TSS) dataset which contains human ranking annotations for the similarity between traffic scenarios. Using the TSS data, we compare our SDL embedding -with textual caption based search methods such as Sentence2Vector. We find that Scenario2Vector outperforms Sentence2Vector by 13% ; and is a promising step towards enabling fair comparisons among AVs by inspecting how they perform in similar traffic situations. We hope that Scenario2Vector can have a similar impact to the AV community that Word2Vec/Sent2Vec have had in Natural Language Processing datasets.}, booktitle = {Proceedings of the ACM/IEEE 12th International Conference on Cyber-Physical Systems}, pages = {167–176}, numpages = {10}, location = {Nashville, Tennessee}, series = {ICCPS '21} } |
|||||
Abstract: A popular metric for measuring progress in autonomous driving has been the "miles per intervention". This is nowhere near a sufficient metric and it does not allow for a fair comparison between the capabilities of two autonomous vehicles (AVs). In this paper we propose Scenario2Vector - a Scenario Description Language (SDL) based embedding for traffic situations that allows us to automatically search for similar traffic situations from large AV data-sets. Our SDL embedding distills a traffic situation experienced by an AV into its canonical components - actors, actions, and the traffic scene. We can then use this embedding to evaluate similarity of different traffic situations in vector space. We have also created a first of its kind, Traffic Scenario Similarity (TSS) dataset which contains human ranking annotations for the similarity between traffic scenarios. Using the TSS data, we compare our SDL embedding -with textual caption based search methods such as Sentence2Vector. We find that Scenario2Vector outperforms Sentence2Vector by 13% ; and is a promising step towards enabling fair comparisons among AVs by inspecting how they perform in similar traffic situations. We hope that Scenario2Vector can have a similar impact to the AV community that Word2Vec/Sent2Vec have had in Natural Language Processing datasets. | |||||
[30] Trent Weiss, Varundev Suresh Babu and Madhur Behl, " Bezier Curve Based End-to-End Trajectory Synthesis for Agile Autonomous Driving", NeurIPS 2020 Machine Learning for Autonomous Driving Workshop | |||||
BibTeX:
@inproceedings{trent2020nips, title={ Bezier Curve Based End-to-End Trajectory Synthesis for Agile Autonomous Driving}, author=Trent Weiss, Varundev Suresh Babu and Madhur Behl}, booktitle={NeurIPS 2020 Machine Learning for Autonomous Driving Workshop}, year={2020} } |
|||||
Abstract: —Demonstrating high-speed autonomous racing can be considered as a grand challenge for vision based end-toend deep learning models. DeepRacing AI is a novel end-to-end framework for trajectory synthesis for autonomous racing. We train and demonstrate the effectiveness of our approach using a high fidelity and photo-realistic Formula One gaming environment - used by real racing drivers. This is the first work that has used the highly realistic F1 game as a simulation environment for deep learning models. We present a novel method for single agent autonomous racing by training a deep neural network to predict a parameterized representation of a trajectory. Our Bezier curve based trajectory synthesis approach outperforms several other end-to-end DNN approaches for autonomous racing. In addition to evaluating our methodology in a closed-loop manner in the game; we also implement the DeepRacing algorithm on a 1/10 scale autonomous racing test-bed and show its ability to handle real-world data at high speeds. | |||||
[29] Trent Weiss, Varundev Suresh Babu and Madhur Behl, "DeepRacing AI: Agile Trajectory Synthesis for Autonomous Racing", International Conference on Intelligent Robotis and Systems [IROS 2020] Workshop on Perception, Learning, and Control for Autonomous Agile Vehicles. [Best Paper Award] | |||||
BibTeX:
@inproceedings{trent2020iros, title={DeepRacing AI: Agile Trajectory Synthesis for Autonomous Racing}, author=Trent Weiss, Varundev Suresh Babu and Madhur Behl}, booktitle={International Conference on Intelligent Robotis and Systems (IROS): Workshop on Perception, Learning, and Control for Autonomous Agile Vehicles.}, year={2020}, organization={IEEE Robotics and Automation Society} } |
|||||
Abstract: —Demonstrating high-speed autonomous racing can be considered as a grand challenge for vision based end-toend deep learning models. DeepRacing AI is a novel end-to-end framework for trajectory synthesis for autonomous racing. We train and demonstrate the effectiveness of our approach using a high fidelity and photo-realistic Formula One gaming environment - used by real racing drivers. This is the first work that has used the highly realistic F1 game as a simulation environment for deep learning models. We present a novel method for single agent autonomous racing by training a deep neural network to predict a parameterized representation of a trajectory. Our Bezier curve based trajectory synthesis approach outperforms several other end-to-end DNN approaches for autonomous racing. In addition to evaluating our methodology in a closed-loop manner in the game; we also implement the DeepRacing algorithm on a 1/10 scale autonomous racing test-bed and show its ability to handle real-world data at high speeds. | |||||
[28] Varundev SureshBabu and Madhur Behl, "F1tenth.dev - an Open-Source ROS Based F1/10 Autonomous Racing Simulator", 2020 IEEE 16th International Conference on Automation Science and Engineering (CASE) | |||||
BibTeX:
@CONFERENCE {varundevsureshbabumadhurbehl2020, author = "Varundev SureshBabu and Madhur Behl", title = "F1tenth.dev - an Open-Source ROS Based F1/10 Autonomous Racing Simulator", booktitle = "IEEE 16th International Conference on Automation Science and Engineering (CASE)", year = "2020", month = "aug", organization = "IEEE" } |
|||||
Abstract: This paper presents the ROS F1/10 simulator- a ROS and Gazebo based autonomous racing simula-tor designed to mirror the behaviour and performanceof the physical F1/10 platform. The simulator can beused to verify the performance of autonomous racingalgorithms before testing on the real F1/10 racecar. Thesimulator supports most major ROS SLAM and navigationpackages with tutorials on how to get started availableon www.f1tenth.dev under open-source license and wecontinue to add support for more algorithms and features.The paper presents details on the simulator architecture,design, and features and presents several research use-cases including an example of developing in the sim andtransferring the performance to the race racecar. | |||||
[27] Hyun Jae Cho and Madhur Behl "Towards Automated Safety Coverage and Testing for Autonomous Vehicles with Reinforcement Learning", 2020 arXiv preprint arXiv:2005.13976 | |||||
BibTeX:
@article{cho2020towards, title={Towards Automated Safety Coverage and Testing for Autonomous Vehicles with Reinforcement Learning}, author={Cho, Hyun Jae and Behl, Madhur}, journal={arXiv preprint arXiv:2005.13976}, year={2020} } |
|||||
Abstract: The kind of closed-loop verification likely to be required for autonomous vehicle (AV) safety testing is beyond the reach of traditional test methodologies and discrete verification. Validation puts the autonomous vehicle system to the test in scenarios or situations that the system would likely encounter in everyday driving after its release. These scenarios can either be controlled directly in a physical (closed-course proving ground) or virtual (simulation of predefined scenarios) environment, or they can arise spontaneously during operation in the real world (open-road testing or simulation of randomly generated scenarios). In AV testing, simulation serves primarily two purposes: to assist the development of a robust autonomous vehicle and to test and validate the AV before release. A challenge arises from the sheer number of scenario variations that can be constructed from each of the above sources due to the high number of variables involved (most of which are continuous). Even with continuous variables discretized, the possible number of combinations becomes practically infeasible to test. To overcome this challenge we propose using reinforcement learning (RL) to generate failure examples and unexpected traffic situations for the AV software implementation. Although reinforcement learning algorithms have achieved notable results in games and some robotic manipulations, this technique has not been widely scaled up to the more challenging real world applications like autonomous driving. | |||||
[26] Trent Weiss and Madhur Behl "DeepRacing: Parameterized Trajectories for Autonomous Racing", 2020 arXiv preprint arXiv:2005.05178 | |||||
BibTeX:
@article{weiss2020deepracing2, title={DeepRacing: Parameterized Trajectories for Autonomous Racing}, author={Weiss, Trent and Behl, Madhur}, journal={arXiv preprint arXiv:2005.05178}, year={2020} } |
|||||
Abstract: We consider the challenging problem of high speed autonomous racing in a realistic Formula One environment. DeepRacing is a novel end-to-end framework, and a virtual testbed for training and evaluating algorithms for autonomous racing. The virtual testbed is implemented using the realistic F1 series of video games, developed by Codemasters, which many Formula One drivers use for training. This virtual testbed is released under an open-source license both as a standalone C++ API and as a binding to the popular Robot Operating System 2 (ROS2) framework. This open-source API allows anyone to use the high fidelity physics and photo-realistic capabilities of the F1 game as a simulator, and without hacking any game engine code. We use this framework to evaluate several neural network methodologies for autonomous racing. Specifically, we consider several fully end-to-end models that directly predict steering and acceleration commands for an autonomous race car as well as a model that predicts a list of waypoints to follow in the car's local coordinate system, with the task of selecting a steering/throttle angle left to a classical control algorithm. We also present a novel method of autonomous racing by training a deep neural network to predict a parameterized representation of a trajectory rather than a list of waypoints. We evaluate these models performance in our open-source simulator and show that trajectory prediction far outperforms end-to-end driving. Additionally, we show that open-loop performance for an end-to-end model, i.e. root-mean-square error for a model's predicted control values, does not necessarily correlate with increased driving performance in the closed-loop sense, i.e. actual ability to race around a track. Finally, we show that our proposed model of parameterized trajectory prediction outperforms both end-to-end control and waypoint prediction. | |||||
[25] Matthew O'Kelly, Varundev Sukhil, Houssam Abbas, Jack Harkins, Chris Kao, Yash Vardhan Pant, Rahul Mangharam, Dipshil Agarwal, Madhur Behl, Paolo Burgio, Marko Bertogna, "F1/10: An open-source autonomous cyber-physical platform", 2019 arXiv preprint arXiv:1901.08567 | |||||
BibTeX:
@article{o2019f1, title={F1/10: An open-source autonomous cyber-physical platform}, author={O'Kelly, Matthew and Sukhil, Varundev and Abbas, Houssam and Harkins, Jack and Kao, Chris and Pant, Yash Vardhan and Mangharam, Rahul and Agarwal, Dipshil and Behl, Madhur and Burgio, Paolo and others}, journal={arXiv preprint arXiv:1901.08567}, year={2019} } |
|||||
Abstract: In 2005 DARPA labeled the realization of viable autonomous vehicles (AVs) a grand challenge; a short time later the idea became a moonshot that could change the automotive industry. Today, the question of safety stands between reality and solved. Given the right platform the CPS community is poised to offer unique insights. However, testing the limits of safety and performance on real vehicles is costly and hazardous. The use of such vehicles is also outside the reach of most researchers and students. In this paper, we present F1/10: an open-source, affordable, and high-performance 1/10 scale autonomous vehicle testbed. The F1/10 testbed carries a full suite of sensors, perception, planning, control, and networking software stacks that are similar to full scale solutions. We demonstrate key examples of the research enabled by the F1/10 testbed, and how the platform can be used to augment research and education in autonomous systems, making autonomy more accessible. | |||||
[24] Gabriel Argush, William Holincheck, Jessica Krynitsky, Brian McGuire, Dax Scott, Charlie Tolleson, Madhur Behl, "Explorer51–Indoor Mapping, Discovery, and Navigation for an Autonomous Mobile Robot", 2020 Systems and Information Engineering Design Symposium (SIEDS), Pages 1-5 | |||||
BibTeX:
@inproceedings{argush2020explorer51, title={Explorer51--Indoor Mapping, Discovery, and Navigation for an Autonomous Mobile Robot}, author={Argush, Gabriel and Holincheck, William and Krynitsky, Jessica and McGuire, Brian and Scott, Dax and Tolleson, Charlie and Behl, Madhur}, booktitle={2020 Systems and Information Engineering Design Symposium (SIEDS)}, pages={1--5}, year={2020}, organization={IEEE} } |
|||||
Abstract: The nexus of robotics, autonomous systems, and artificial intelligence (AI) has the potential to change the nature of human guided exploration of indoor and outdoor spaces. Such autonomous mobile robots can be incorporated into a variety of applications, ranging from logistics and maintenance, to intelligence gathering, surveillance, and reconnaissance (ISR). One such example is that of a tele-operator using the robot to generate a map of the inside of a building while discovering and tagging the objects of interest. During this process, the tele-operator can also assign an area for the robot to navigate autonomously or return to a previously marked area/object of interest. Search and rescue and ISR abilities could be immensely improved with such capabilities. The goal of this research is to prototype and demonstrate the above autonomous capabilities in a mobile ground robot called Explorer51. Objectives include: (i) enabling an operator to drive the robot non-line of sight to explore a space by incorporating a first-person view (FPV) system to stream data from the robot to the base station; (ii) implementing automatic collision avoidance to prevent the operator from running the robot into obstacles; (iii) creating and saving 2D and 3D maps of the space in real time by using a 2D laser scanner, tracking, and depth/RGB cameras; (iv) locating and tagging objects of interest as waypoints within the map; (v) autonomously navigate within the map to reach a chosen waypoint. To accomplish these goals, we are using the AION Robotics R1 Unmanned Ground Vehicle (UGV) rover as the platform for Explorer51 to demonstrate the autonomous features. The rover runs the Robot Operating System (ROS) onboard an NVIDIA Jetson TX2 board, connected to a Pixhawk controller. Sensors include a 2D scanning LiDAR, depth camera, tracking camera, and an IMU. Using existing ROS packages such as Cartographer and TEB planner, we plan to implement ROS nodes for accomplishing these tasks. We plan to extend the mapping ability of the rover using Visual Inertial Odometry (VIO) using the cameras. In addition, we will explore the implementation of additional features such as autonomous target identification, waypoint marking, collision avoidance, and iterative trajectory optimization. The project will culminate in a series of demonstrations to showcase the autonomous navigation, and tele-operation abilities of the robot. Success will be evaluated based on ease of use by the tele-operator, collision avoidance ability, autonomous waypoint navigation accuracy, and robust map creation at high driving speeds. | |||||
[23] Trent Weiss, Madhur Behl, "DeepRacing: a framework for autonomous racing", 2020 IEEE Design, Automation & Test in Europe Conference & Exhibition (DATE), Pages 1163-1168 | |||||
BibTeX:
@inproceedings{weiss2020deepracing, title={DeepRacing: a framework for autonomous racing}, author={Weiss, Trent and Behl, Madhur}, booktitle={2020 Design, Automation \& Test in Europe Conference \& Exhibition (DATE)}, pages={1163--1168}, year={2020}, organization={IEEE} } |
|||||
Abstract: We consider the challenging problem of high speed autonomous racing in realistic dynamic environments. DeepRacing is a novel end-to-end framework, and a virtual testbed for training and evaluating algorithms for autonomous racing. The virtual testbed is implemented using the realistic Formula One (F1) Codemasters game, which is used by many F1 drivers for training. We present AdmiralNet - a Convolution Neural Network (CNN) integrated with Long Short-Term Memory (LSTM) cells that can be tuned for the autonomous racing task in the highly realistic F1 game. We evaluate AdmiralNet's performance on unseen race tracks, and also evaluate the degree of transference between the simulation and the real world by implementing end-to-end racing on a physical 1/10 scale autonomous racecar. | |||||
[22] Madhur Behl, Jackson DuBro, Taylor Flynt, Imaan Hameed, Grace Lang, Felix Park, "Autonomous Electric Vehicle Charging System", 2019 Systems and Information Engineering Design Symposium (SIEDS), Pages 1-6 [Best Systems Design Award] | |||||
BibTeX:
@inproceedings{behl2019autonomous, title={Autonomous Electric Vehicle Charging System}, author={Behl, Madhur and DuBro, Jackson and Flynt, Taylor and Hameed, Imaan and Lang, Grace and Park, Felix}, booktitle={2019 Systems and Information Engineering Design Symposium (SIEDS)}, pages={1--6}, year={2019}, organization={IEEE} } |
|||||
Abstract: Electric vehicle (EV) adoption has surpassed the growth of charging infrastructure. As the demand for charging stations surpass the supply, expanding charging infrastructure for consumers is crucial to improving the experience of owning and maintaining an EV. One solution is to simply provide more charging stations; however, this requires significant upfront hardware and space cost. In addition, parking spots allocated for EVs should only be used by EVs, forcing manufacturers to make a decision on the number of EV and non-EV parking spots. Current charging stations also have their own problems. When an EV is finished charging, any additional time it spends in the charging location is time that another EV could be using to charge itself. Innovative new products are necessary to create an adequate charging network. In this work, a mobile autonomous robot which charges parked EVs at any location with its own battery is presented. We created a proof-of-concept autonomous charging robot to demonstrate feasibility and motivate future work. The goal is to provide three main decoupled functionalities: parking lot navigation, EV plug guidance, and robot battery swapping. The current iteration meets these functionalities using a TurtleBot to navigate a mock parking lot, new designs and prototypes for swapping batteries, and a robotic arm paired with a computer vision algorithm to guide a 3D printed plug. Ongoing challenges for future iterations involve integrating the main functionalities and dealing with a wider range of less common use cases. | |||||
[21] Alexander B Chen, Madhur Behl, Jonathan L Goodall, "Trust me, my neighbors say it's raining outside: ensuring data trustworthiness for crowdsourced weather stations", 2018 Proceedings of the 5th Conference on Systems for Built Environments, Pages 25-28 [Best Presentation Award] | |||||
BibTeX:
@inproceedings{chen2018trust, title={Trust me, my neighbors say it's raining outside: ensuring data trustworthiness for crowdsourced weather stations}, author={Chen, Alexander B and Behl, Madhur and Goodall, Jonathan L}, booktitle={Proceedings of the 5th Conference on Systems for Built Environments}, pages={25--28}, year={2018} } |
|||||
Abstract: Decision making in utilities, municipal, and energy companies depends on accurate and trustworthy weather information and predictions. Recently, crowdsourced personal weather stations (PWS) are being increasingly used to provide a higher spatial and temporal resolution of weather measurements. However, tools and methods to ensure the trustworthiness of the crowdsourced data in real-time are lacking. In this paper, we present a Reputation System for Crowdsourced Rainfall Networks (RSCRN) to assign trust scores to personal weather stations in a region. Using real PWS data from the Weather Underground service in the high flood risk region of Norfolk, Virginia, we evaluate the performance of the proposed RSCRN. The proposed method is able to converge to a confident trust score for a PWS within 10--20 observations after installation. Collectively, the results indicate that the trust score derived from the RSCRN can reflect the collective measure of trustworthiness to the PWS, ensuring both useful and trustworthy data for modeling and decision-making in the future. | |||||
[20] Alexander B Chen, Madhur Behl, Jonathan L Goodall, "Trust me, my neighbors say it's raining outside: ensuring data trustworthiness for crowdsourced weather stations", 2018 Proceedings of the 5th Conference on Systems for Built Environments, Pages 25-28 | |||||
BibTeX:
@inproceedings{chen2018trust, title={Trust me, my neighbors say it's raining outside: ensuring data trustworthiness for crowdsourced weather stations}, author={Chen, Alexander B and Behl, Madhur and Goodall, Jonathan L}, booktitle={Proceedings of the 5th Conference on Systems for Built Environments}, pages={25--28}, year={2018} } |
|||||
Abstract: Decision making in utilities, municipal, and energy companies depends on accurate and trustworthy weather information and predictions. Recently, crowdsourced personal weather stations (PWS) are being increasingly used to provide a higher spatial and temporal resolution of weather measurements. However, tools and methods to ensure the trustworthiness of the crowdsourced data in real-time are lacking. In this paper, we present a Reputation System for Crowdsourced Rainfall Networks (RSCRN) to assign trust scores to personal weather stations in a region. Using real PWS data from the Weather Underground service in the high flood risk region of Norfolk, Virginia, we evaluate the performance of the proposed RSCRN. The proposed method is able to converge to a confident trust score for a PWS within 10--20 observations after installation. Collectively, the results indicate that the trust score derived from the RSCRN can reflect the collective measure of trustworthiness to the PWS, ensuring both useful and trustworthy data for modeling and decision-making in the future. | |||||
[19] Arash Tavakoli, Amir Ashrafi, Arsalan Heydarian, Madhur Behl, "The internet of wasted things (IoWT)", Proceedings of the 8th International Conference on the Internet of Things, Pgs 1-3 | |||||
BibTeX:
@inproceedings{tavakoli2018internet, title={The internet of wasted things (IoWT)}, author={Tavakoli, Arash and Ashrafi, Amir and Heydarian, Arsalan and Behl, Madhur}, booktitle={Proceedings of the 8th International Conference on the Internet of Things}, pages={1--3}, year={2018} } |
|||||
Abstract: Office buildings, schools, stores, hotels, restaurants and other commercial, and institutional buildings generate significant amounts of waste. While buildings are becoming increasingly aware of their energy and water usage, the capability to track waste and material use with the same ease has remained beyond the reach of most building facilities managers [1,2]. Studies have focused on the use of sensors for garbage collection at a city level [3]. However, these studies consider waste as bulk and do not itemize and differentiate between their types and amounts [4]. In addition, to achieve the recycling goals, the facilities management offices have to manually or semi-automatically sort through the waste in order to separate the recycle waste apart manually. | |||||
[18] Jeffrey M Sadler, Jonathan L Goodall, Madhur Behl, Mohamed M Morsy, "Leveraging Open Source Software and Parallel Computing for Model Predictive Control Simulation of Urban Drainage Systems Using EPA-SWMM5 and Python", Proceedings of the 2018 International Conference on Urban Drainage Modelling. Pages 988-992 | |||||
BibTeX:
@inproceedings{sadler2018leveraging2, title={Leveraging Open Source Software and Parallel Computing for Model Predictive Control Simulation of Urban Drainage Systems Using EPA-SWMM5 and Python}, author={Sadler, Jeffrey M and Goodall, Jonathan L and Behl, Madhur and Morsy, Mohamed M}, booktitle={International Conference on Urban Drainage Modelling}, pages={988--992}, year={2018}, organization={Springer} } |
|||||
Abstract: The active control of stormwater systems is a potential solution to increased street flooding in low-lying, low-relief coastal cities due to climate change and accompanying sea level rise. Model predictive control (MPC) has been shown to be a successful control strategy generally and as well as for managing urban drainage specifically. This research describes and demonstrates the implementation of MPC for urban drainage systems using open source software (Python and The United States Environmental Protection Agency (EPA) Storm Water Management Model (SWMM5). The system was demonstrated using a simplified use case in which an actively-controlled outlet of a detention pond is simulated. The control of the pond’s outlet influences the flood risk of a downstream node. For each step in the SWMM5 model, a series of policies for controlling the outlet are evaluated. The best policy is then selected using an evolutionary algorithm. The policies are evaluated against an objective function that penalizes primarily flooding and secondarily deviation of the detention pond level from a target level. Freely available Python libraries provide the key functionality for the MPC workflow: step-by-step running of the SWMM5 simulation, evolutionary algorithm implementation, and leveraging parallel computing. For perspective, the MPC results were compared to results from a rule-based approach and a scenario with no active control. The MPC approach produced a control policy that largely eliminated flooding (unlike the scenario with no active control) and maintained the detention pond’s water level closer to a target level (unlike the rule-based approach). | |||||
[17] Achin Jain, Madhur Behl, and Rahul Mangharam (2017), "Data Predictive Control for building energy management.", Proceedings of the 2017 American Control Conference (ACC), Seattle, WA, USA. [Best Energy Systems Paper Award] | |||||
BibTeX:
@conference{DpcACC17, author = {Achin Jain and Madhur Behl and Rahul Mangharam}, title = {Data Predictive Control for building energy management}, journal = {Proceedings of the American Control Conference}, year = {2017}, note = {[Best Energy Systems Paper Award]}, url = {http://repository.upenn.edu/cgiviewcontent.cgi?article=1115&context=mlab_papers} } |
|||||
Abstract: Decisions on how to best optimize energy systems operations are becoming ever so complex and conflicting, that model-based predictive control (MPC) algorithms must play an important role. However, a key factor prohibiting the widespread adoption of MPC in buildings, is the cost, time, and effort associated with learning first-principles based dynamical models of the underlying physical system. This paper introduces an alternative approach for implementing finite-time receding horizon control using control-oriented data-driven models. We call this approach Data Predictive Control (DPC). Specifically, by utilizing separation of variables, two novel algorithms for implementing DPC using a single regression tree and with regression trees ensembles (random forest) are presented. The data predictive controller enables the building operator to trade off energy consumption against thermal comfort without having to learn white/grey box models of the systems dynamics. We present a comprehensive numerical study which compares the performance of DPC with an MPC based energy management strategy, using a single zone building model. Our results demonstrate that performance of DPC is comparable to an MPC controller, with only 3.8% additional cost in terms of optimal objective function and within 95% in terms of R2 score, thereby making it an alluring alternative to MPC, whenever the associated cost of learning the model is high. | |||||
[16] Achin Jain, Rahul Mangharam, and Madhur Behl (2016), "Data Predictive Control for Peak Power Reduction.", 3rd ACM International Conference on Embedded Systems For Energy-Efficient Built Environments (BuildSys), Stanford, CA, USA. [Best Presentation Award] | |||||
BibTeX:
@inproceedings{Jain:2016:DPC:2993422.2993582, author = {Jain, Achin and Mangharam, Rahul and Behl, Madhur}, title = {Data Predictive Control for Peak Power Reduction}, booktitle = {Proceedings of the 3rd ACM International Conference on Systems for Energy-Efficient Built Environments}, series = {BuildSys '16}, year = {2016}, isbn = {978-1-4503-4264-3}, location = {Palo Alto, CA, USA}, pages = {109--118}, numpages = {10}, url = {http://doi.acm.org/10.1145/2993422.2993582}, doi = {10.1145/2993422.2993582}, acmid = {2993582}, publisher = {ACM}, address = {New York, NY, USA}, keywords = {Building control, Machine learning, Peak power reduction, Predictive control}, } |
|||||
Abstract: Decisions on how best to optimize today's energy systems operations are becoming ever so complex and conflicting such that model-based predictive control algorithms must play a key role. However, learning dynamical models of energy consuming systems such as buildings, using grey/white box approaches is very cost and time prohibitive due to its complexity. This paper presents data-driven methods for making control-oriented model for peak power reduction in buildings. Specifically, a data predictive control with regression trees (DPCRT) algorithm, is presented. DPCRT is a finite receding horizon method, using which the building operator can optimally trade off peak power reduction against thermal comfort without having to learn white/grey box models of the systems dynamics. We evaluate the performance of our method using a DoE commercial reference virtual test-bed and show how it can be used for learning predictive models with 90% accuracy, and for achieving 8.6% reduction in peak power and costs. | |||||
[15] Rahul Mangharam, Houssam Abbas, Madhur Behl, Kuk Jang, Miroslav Pajic, Zhihao Jiang (2016), "Three challenges in cyber-physical systems", 8th International Conference on Communication Systems and Networks (COMSNETS), 2016 Vienna, Austria | |||||
BibTeX:
@inproceedings{mangharam2016three, title={Three challenges in cyber-physical systems}, author={Mangharam, Rahul and Abbas, Houssam and Behl, Madhur and Jang, Kuk and Pajic, Miroslav and Jiang, Zhihao}, booktitle={Communication Systems and Networks (COMSNETS), 2016 8th International Conference on}, pages={1--8}, year={2016}, organization={IEEE} } |
|||||
Abstract: The tight coupling of computation, communication and control with physical systems such as actuation of closed-loop medical devices within the human body, peak power minimization by coordination of controllers across large industrial plants, and fast life-critical decision making by autonomous vehicles, present a set of fundamental and unique challenges. Each of these require new approaches at the intersection of multiple scientific, human and systems disciplines. We discuss five such challenges which require creative insights and application of model-based design, control systems, scheduling theory, formal methods, statistical machine learning and domain-specific experimentation. We ask the following questions: (1) An autonomous medical device is implanted to control your heart over a period of 5-7 years. How do you guarantee the software in the device provides safe and effective treatment under all physiological conditions? (2) Electricity prices in the US have summer peaks that are over 32× their average prices and winter peaks that are 86×. How can buildings respond to massive swings in energy prices at fast time scales? (3) While wireless has been successfully used for open-loop monitoring and tracking, how can we operate closed-loop control systems over a network of wireless controllers. Furthermore, how can we ensure robust, optimal and secure control in the presence of node/link failures and topology changes? | |||||
[14] Madhur Behl, Achin Jain and Rahul Mangharam (2016), "Data-Driven Modeling, Control and Tools for Cyber-Physical Energy Systems", International Conference on Cyber-Physical Systems (ICCPS), Vienna, Austria. | |||||
BibTeX:
@conference{behl_iccps16, author = {Madhur Behl and Achin Jain and Rahul Mangharam}, title = {Data-Driven Modeling, Control and Tools for Cyber-Physical energy Systems}, journal = {International Conference on Cyber-Physical Systems (ICCPS)}, year = {2016}, note = {[To Appear]}, url = {http://repository.upenn.edu/mlab_papers/80/} } |
|||||
Abstract: Demand response (DR) is becoming increasingly important as the volatility on the grid continues to increase. Current DR approaches are completely manual and rule-based or involve deriving first principles based models which are extremely cost and time prohibitive to build. We consider the problem of data-driven end-user DR for large buildings which involves predicting the demand response baseline, evaluating fixed rule based DR strategies and synthesizing DR control actions. We provide a model based control with regression trees algorithm (mbCRT), which allows us to perform closed-loop control for DR strategy synthesis for large commercial buildings. Our data-driven control synthesis algorithm outperforms rule-based DR by 17% for a large DoE commercial reference building and leads to a curtailment of 380kW and over $45,000 in savings. Our methods have been integrated into an open source tool called DR-Advisor, which acts as a recommender system for the building's facilities manager and provides suitable control actions to meet the desired load curtailment while maintaining operations and maximizing the economic reward. DR-Advisor achieves 92.8% to 98.9% prediction accuracy for 8 buildings on Penn's campus. We compare DR-Advisor with other data driven methods and rank 2nd on ASHRAE's benchmarking data-set for energy prediction. | |||||
[13] Madhur Behl and Rahul Mangharam (2015), "Sometimes, Money Does Grow On Trees: Real-Time Demand Response With DR-Advisor", 2nd ACM International Conference on Embedded Systems For Energy-Efficient Built Environments (BuildSys), Seoul, South Korea. , pp. 137-146. | |||||
BibTeX:
@conference{behl_buildsys15, author = {Madhur Behl and Rahul Mangharam}, title = {Sometimes, Money Does Grow On Trees: Real-Time Demand Response With DR-Advisor}, journal = {2nd ACM International Conference on Embedded Systems For Energy-Efficient Built Environments (BuildSys), Seoul, South Korea}, year = {2015}, pages = {137-146}, url = {http://repository.upenn.edu/mlab_papers/82/}, doi = {10.1145/2821650.2821664} } |
|||||
Abstract: Real-time electricity pricing and demand response has be- come a clean, reliable and cost-effective way of mitigating peak demand on the electricity grid. We consider the problem of end-user demand response (DR) for large commer- cial buildings which involves predicting the demand response baseline, evaluating fixed DR strategies and synthesizing DR control actions for load curtailment in return for a financial reward. Using historical data from the building, we build a family of regression trees and learn data-driven models for predicting the power consumption of the building in real-time. We present a method called DR-Advisor called DR- Advisor, which acts as a recommender system for the build- ing’s facilities manager and provides suitable control actions to meet the desired load curtailment while maintaining op- erations and maximizing the economic reward. We evaluate the performance of DR-Advisor for demand response using data from a real office building and a virtual test-bed. | |||||
[12] Madhur Behl and Rahul Mangharam (2015), "Sometimes, Money Does Grow on Trees: DR-Advisor, A Data Driven Demand Response Recommender System", Semiconductor Research Corporation (SRC) TECHCON. Vol. Publication ID:P084437, Austin, USA. [Best Paper Award] | |||||
BibTeX:
@conference{behl_techcon, author = {Madhur Behl and Rahul Mangharam}, title = {Sometimes, Money Does Grow on Trees: DR-Advisor, A Data Driven Demand Response Recommender System}, journal = {Semiconductor Research Corporation (SRC) TECHCON}, year = {2015}, volume = {Publication ID:P084437}, note = {Best Paper Award in the Internet of Things Session}, url = {http://repository.upenn.edu/mlab_papers/81/} } |
|||||
Abstract: Unprecedented amounts of information from mil- lions of smart meters and thermostats installed in recent years has left the door open for better understanding, analyzing and using the insights that data can provide, about the power consumption patterns of a building. The challenge with using data-driven approaches, is to close the loop for near real-time control and decision making in large buildings. Furthermore, providing a technological solution alone is not enough, the solution must also be human centric. We consider the problem of end-user demand response for commercial buildings. Using historical data from the building, we build a family of regression trees based models for predicting the power consumption of the building in real-time. We have built DR-Advisor, a recommender system for the building’s facilities manager, which provides optimal control actions to meet the required load curtailment while maintaining building operations and maximizing the economic reward. | |||||
[11] Madhur Behl, Truong Nghiem and Rahul Mangharam (2015), "DR-Advisor: A Data Driven Demand Response Recommender System", Proceedings of International Conference CISBAT: Future Buildings and Districts Sustainability from Nano to Urban Scale. Vol. EPFL-CONF-213354, pp. 401-406. Lausane, Switzerland. | |||||
BibTeX:
@proceedings{behl_cisbat, author = {Madhur Behl and Truong Nghiem and Rahul Mangharam}, title = {DR-Advisor: A Data Driven Demand Response Recommender System}, journal = {Proceedings of International Conference CISBAT: Future Buildings and Districts Sustainability from Nano to Urban Scale}, year = {2015}, volume = {EPFL-CONF-213354}, pages = {401-406}, url = {http://repository.upenn.edu/mlab_papers/78/} } |
|||||
Abstract: A data-driven method for demand response baselining and strategy evaluation is pre- sented. Using meter and weather data along with set-point schedule information, we use an ensemble of regression trees to learn non-parametric data-driven models for predicting the power consumption of the building. This model can be used for evaluating demand response strategies in real-time, without having to learn complex models of the building. The methods have been integrated in an open-source tool called DR-Advisor, which acts as a recommender system for the building’s facilities manager by advising on which con- trol actions should be during a demand response event. We provide a case study using data from a large commercial vistural test-bed building to evaluate the performance of the DR-Advisor tool. | |||||
[10] Willy Bernal, Madhur Behl, Truong Nghiem and Rahul Mangharam (2015), "Campus-Wide Integrated Building Energy Simulation", 14th International Conference of the International Building Performance Simulation Association. Hyderabad, India. | |||||
BibTeX:
@conference{bernal_ibpsa, author = {Willy Bernal and Madhur Behl and Truong Nghiem and Rahul Mangharam}, title = {Campus-Wide Integrated Building Energy Simulation}, journal = {14th International Conference of the International Building Performance Simulation Association}, year = {2015}, url = {http://repository.upenn.edu/mlab_papers/85/} } |
|||||
Abstract: Effective energy management for large campus facilities is becoming increasingly complex as modern heat- ing and cooling systems comprise of several hundred subsystems interconnected to each other. Building energy simulators like EnergyPlus are exceedingly good at modeling a single building equipped with a standalone HVAC equipment. However, the ability to simulate a large campus and to control the dynamics and interactions of the subsystems is limited or missing al- together. In this paper, we use the Matlab-EnergyPlus MLE+ tool we developed, to extend the capability of EnergyPlus to co-simulate a campus with multiple build- ings connected to a chilled water distribution to a central chiller plant with control systems in Matlab. We present the details of how this simulation can be set- up and implemented using MLE+’s Matlab/Simulink block. We utilize the virtual campus test-bed to evaluate the performance of several demand response strate- gies. We also describe a coordinated demand response scheme which can lead to load curtailment during a demand response event while minimizing thermal discomfort. |
|||||
[9] Madhur Behl, Truong Nghiem and Rahul Mangharam (2014), "IMpACT Inverse Model Accuracy and Control Performance Toolbox for Buildings", 2014 IEEE International Conference on Automation Science and Engineering (CASE). , pp. 1109-1114. Taipei, Taiwan. | |||||
BibTeX:
@conference{behl_case14, author = {Madhur Behl and Truong Nghiem and Rahul Mangharam}, title = {IMpACT Inverse Model Accuracy and Control Performance Toolbox for Buildings}, journal = {2014 IEEE International Conference on Automation Science and Engineering (CASE)}, year = {2014}, pages = {1109-1114}, url = {http://repository.upenn.edu/cgi/viewcontent.cgi?article=1083&context=mlab_papers} } |
|||||
Abstract: Uncertainty a ects all aspects of building performance: from the identi cation of models, through the implementation of model-based control, to the operation of the deployed systems. Learning models of buildings from sensor data has a fundamental property that the model can only be as accurate and reliable as the data on which it was trained. For small and medium size buildings, a low-cost method for model capture is necessary to take advantage of optimal model-based supervisory control schemes. We present IMpACT, a methodology and a toolbox for analysis of uncertainty propagation for building inverse modeling and controls. Given a plant model and real input data, IMpACT automatically evaluates the e ect of the uncertainty propagation from sensor data to model accuracy and control performance. We also present a statistical method to quantify the bias in the sensor measurement and to determine near optimal sensor placement and density for accurate signal measurements. In our previous work, we considered the end-to-end propagation of uncertainty in the form of xed bias in the sensor data. In this paper, we extend the method to work with random errors in the sensor data, which is more realistic. Using a real building test-bed, we show how performing an uncertainty analysis can reveal trends about inverse model accuracy and control performance, which can be used to make informed decisions about sensor requirements and data accuracy. | |||||
[8] Madhur Behl, Truong Nghiem and Rahul Mangharam (2014), "Model-IQ: Uncertainty Propagation from Sensing to Modeling and Control in Buildings.", ACM/IEEE 5th International Conference on Cyber-Physical Systems. Berlin, Germany. | |||||
BibTeX:
@conference{behl_iccps14, author = {Madhur Behl and Truong Nghiem and Rahul Mangharam}, title = {Model-IQ: Uncertainty Propagation from Sensing to Modeling and Control in Buildings.}, journal = {ACM/IEEE 5th International Conference on Cyber-Physical Systems}, year = {2014}, url = {http://repository.upenn.edu/cgi/viewcontent.cgi?article=1082&context=mlab_papers} } |
|||||
Abstract: A fundamental problem in the design of closed-loop Cyber-Physical Systems (CPS) is in accurately capturing the dynamics of the underlying physical system. To provide optimal control for such closed-loop systems, model-based controls require accurate physical plant models. It is hard to analytically establish (a) how data quality from sensors affects model accuracy, and consequently, (b) the effect of model accuracy on the operational cost of model-based controllers. We present the Model-IQ toolbox which, given a plant model and real input data, automatically evaluates the effect of this uncertainty propagation from sensor data to model accuracy to controller performance. We apply the Model-IQ uncertainty analysis for model-based controls in buildings to demonstrate the cost-benefit of adding temporary sensors to capture a building model. We show how sensor placement and density bias training data. For the real building considered, a bias of 1% degrades model accuracy by 20%. Model-IQ's automated process lowers the cost of sensor deployment, model training and evaluation of advanced controls for small and medium sized buildings. Such end-to-end analysis of uncertainty propagation has the potential to lower the cost for CPS with closed-loop model based control. We demonstrate this with real building data in the Department of Energy's HUB. |
|||||
[7] Madhur Behl, Truong Nghiem and Rahul Mangharam (2012), "Green Scheduling for Energy-Efficient Operation of Multiple Chiller Plants", In IEEE 33rd Real-Time Systems Symposium (RTSS 2012). , pp. 195 - 204. San Juan, Puerto Rico. | |||||
BibTeX:
@inproceedings{BehlEtAl12rtss, author = {Madhur Behl and Truong Nghiem and Rahul Mangharam}, title = {Green Scheduling for Energy-Efficient Operation of Multiple Chiller Plants}, booktitle = {IEEE 33rd Real-Time Systems Symposium (RTSS 2012)}, year = {2012}, pages = {195 - 204}, url = {http://ieeexplore.ieee.org/xpl/login.jsp?tp=&arnumber=6424803&url=http%3A%2F%2Fieeexplore.ieee.org%2Fxpls%2Fabs_all.jsp%3Farnumber%3D6424803}, doi = {10.1109/RTSS.2012.71} } |
|||||
Abstract: In large building systems, such as a university campus, the air-conditioning systems are commonly served by chiller plants, which contribute a large fraction of the total elec- tricity consumption of the campuses. The power consumption of a chiller is highly affected by its Coefficient of Performance (COP), which is optimal when the chiller is operated at or near full load. For a chiller plant, its overall COP can be optimized by utilizing a Thermal Energy Storage (TES) and switching its operation between COP-optimal charging and discharging modes. However, uncoordinated mode switchings of chiller plants may cause temporally-correlated high electricity demand when multiple plants are charging their TES concurrently. In this paper, a Green Scheduling approach, proposed in our previous work, is used to schedule the chiller plants to reduce their peak aggregate power demand while ensuring safe operation of the TES. We present a scheduling algorithm based on backward reach set computation of the TES dynamics. The proposed algorithm is demonstrated in a numerical simulation in Matlab to be effective for reducing the peak power demand and the overall electricity cost. | |||||
[6] Truong Nghiem, Madhur Behl, George Pappas and Rahul Mangharam (2012), "Green scheduling for radiant systems in buildings", In IEEE 51st Annual Conference on Decision and Control (CDC). , pp. 7577-7582. IEEE. Maui, Hawaii. | |||||
BibTeX:
@conference{NghiemEtAl12cdc, author = {Truong Nghiem and Madhur Behl and George Pappas and Rahul Mangharam}, title = {Green scheduling for radiant systems in buildings}, booktitle = {IEEE 51st Annual Conference on Decision and Control (CDC)}, publisher = {IEEE}, year = {2012}, pages = {7577-7582}, url = {http://ieeexplore.ieee.org/xpl/login.jsp?tp=&arnumber=6426318&url=http%3A%2F%2Fieeexplore.ieee.org%2Fxpls%2Fabs_all.jsp%3Farnumber%3D6426318}, doi = {10.1109/CDC.2012.6426318} } |
|||||
Abstract: In this paper we look at the problem of peak power reduction for buildings with electric radiant floor heating systems. Uncoordinated operation of a multi-zone radiant floor heating system can result in temporally correlated electricity demand surges or peaks in the building's electricity consumption. As peak power prices are 200-400 times that of the nominal rate, this uncoordinated activity can result in high electricity costs and expensive system operation. We have previously presented green scheduling as an approach for reducing the aggregate peak power consumption in buildings while ensuring that indoor thermal comfort is always maintained. This paper extends the theoretical results for general affine dynamical systems and applies them to electric radiant floor heating systems. The potential of the proposed method in reducing the peak power demand is demonstrated for a small-scale system through simulation in EnergyPlus and for a large-scale system through simulation in Matlab. | |||||
[5] Willy Bernal, Madhur Behl, Truong Nghiem and Rahul Mangharam (2012), "MLE+: a tool for integrated design and deployment of energy efficient building controls", In BuildSys '12 Proceedings of the Fourth ACM Workshop on Embedded Sensing Systems for Energy-Efficiency in Buildings. , pp. 123-130. Toronto, Canada. | |||||
BibTeX:
@proceedings{BernalEtAl12buildsys, author = {Willy Bernal and Madhur Behl and Truong Nghiem and Rahul Mangharam}, title = {MLE+: a tool for integrated design and deployment of energy efficient building controls}, booktitle = {BuildSys '12 Proceedings of the Fourth ACM Workshop on Embedded Sensing Systems for Energy-Efficiency in Buildings}, publisher = {ACM New York, NY, USA ©2012}, year = {2012}, pages = {123-130}, note = {Best Demo Award}, url = {http://dl.acm.org/citation.cfm?id=2422553}, doi = {10.1145/2422531.2422553} } |
|||||
Abstract: We present MLE+, a tool for energy-efficient building automation design, co-simulation and analysis. The tool leverages the high-fidelity building simulation capabilities of EnergyPlus and the scientific computation and design capabilities of Matlab for controller design. MLE+ facilitates integrated building simulation and controller formulation with integrated support for system identification, control design, optimization, simulation analysis and communication between software applications and building equipment. It provides streamlined workflows, a graphical front-end, and debugging support to help control engineers eliminate design and programming errors and take informed decisions early in the design stage, leading to fewer iterations in the building automation development cycle. We show through an example and two case studies how MLE+ can be used for designing energy-efficient control algorithms for both simulated buildings in EnergyPlus and real building equipment via BACnet. | |||||
[4] Truong Nghiem, Madhur Behl, Rahul Mangharam and George Pappas (2012), "Scalable scheduling of building control systems for peak demand reduction", In American Control Conference (ACC),. , pp. 3050 - 3055. Montreal, Canada. | |||||
BibTeX:
@conference{acc12, author = {Truong Nghiem and Madhur Behl and Rahul Mangharam and George Pappas}, title = {Scalable scheduling of building control systems for peak demand reduction}, booktitle = {American Control Conference (ACC),}, publisher = {IEEE}, year = {2012}, pages = {3050 - 3055}, url = {http://ieeexplore.ieee.org/xpl/login.jsp?tp=&arnumber=6315252&url=http%3A%2F%2Fieeexplore.ieee.org%2Fxpls%2Fabs_all.jsp%3Farnumber%3D6315252}, doi = {10.1109/ACC.2012.6315252} } |
|||||
Abstract: In large energy systems, peak demand might cause severe issues such as service disruption and high cost of energy production and distribution. Under the widely adopted peak-demand pricing policy, electricity customers are charged a very high price for their maximum demand to discourage their energy usage in peak load conditions. In buildings, peak demand is often the result of temporally correlated energy demand surges caused by uncoordinated operation of subsystems such as heating, ventilating, air conditioning and refrigeration (HVAC&R) systems and lighting systems. We have previously presented green scheduling as an approach to schedule the building control systems within a constrained peak demand envelope while ensuring that custom climate conditions are facilitated. This paper provides a sufficient schedulability condition for the peak constraint to be realizable for a large and practical class of system dynamics that can capture certain nonlinear dynamics, inter-dependencies, and constrained disturbances. We also present a method for synthesizing periodic schedules for the system. The proposed method is demonstrated in a simulation example to be scalable and effective for a large-scale system. | |||||
[3] Truong X. Nghiem, Madhur Behl, Rahul Mangharam and George J. Pappas (2011), "Green scheduling of control systems for peak demand reduction", In 50th IEEE Conference on Decision and Control and European Control Conference (CDC-ECC),. , pp. 5131 - 5136. IEEE. Orlando, USA | |||||
BibTeX:
@conference{NghiemEtAl11gso, author = {Truong X. Nghiem and Madhur Behl and Rahul Mangharam and George J. Pappas}, title = {Green scheduling of control systems for peak demand reduction}, booktitle = {50th IEEE Conference on Decision and Control and European Control Conference (CDC-ECC),}, publisher = {IEEE}, year = {2011}, pages = {5131 - 5136}, url = {http://repository.upenn.edu/cgi/viewcontent.cgi?article=1065&context=mlab_papers}, doi = {10.1109/CDC.2011.6161164} } |
|||||
Abstract: Building systems such as heating, air quality control and refrigeration operate independently of each other and frequently result in temporally correlated energy demand surges. As peak power prices are 200-400 times that of the nominal rate, this uncoordinated activity is both expensive and operationally inefficient. We present an approach to fine-grained coordination of energy demand by scheduling the control systems within a constrained peak while ensuring custom climate environments are facilitated. The peak constraint is minimized for energy efficiency, while we provide feasibility conditions for the constraint to be realizable by a scheduling policy for the control systems. The physical systems are then coordinated by the scheduling controller so as both the peak constraint and the climate/safety constraint are satisfied. We also introduce a simple scheduling approach called lazy scheduling. The proposed control and scheduling strategy is implemented in simulation examples from small to large scales, which show that it can achieve significant peak demand reduction while being efficient and scalable. | |||||
[2] Zheng Li, Pei-Chi Huang, Aloysius Mok, Truong Nghiem, Madhur Behl, George Pappas and Rahu Mangharam (2011), "On the feasibility of linear discrete-time systems of the green scheduling problem", In IEEE 32nd Real-Time Systems Symposium (RTSS),. , pp. 295 -304. Vienna, Austria. | |||||
BibTeX:
@inproceedings{6121447, author = { Zheng Li and Pei-Chi Huang and Aloysius Mok and Truong Nghiem and Madhur Behl and George Pappas and Rahul Mangharam}, title = {On the feasibility of linear discrete-time systems of the green scheduling problem}, booktitle = {IEEE 32nd Real-Time Systems Symposium (RTSS),}, publisher = {IEEE}, year = {2011}, pages = {295 -304}, url = {http://georgejpappas.org/papers/06121447.pdf}, doi = {10.1109/RTSS.2011.34} } |
|||||
Abstract: Peak power consumption of buildings in large facilities like hospitals and universities becomes a big issue because peak prices are much higher than normal rates. During a power demand surge an automated power controller of a building may need to schedule ON and OFF different environment actuators such as heaters and air quality control while maintaining the state variables such as temperature or air quality of any room within comfortable ranges. The green scheduling problem asks whether a scheduling policy is possible for a system and what is the necessary and sufficient condition for systems to be feasible. In this paper we study the feasibility of the green scheduling problem for HVAC(Heating, Ventilating, and Air Conditioning) systems which are approximated by a discrete-time model with constant increasing and decreasing rates of the state variables. We first investigate the systems consisting of two tasks and find the analytical form of the necessary and sufficient conditions for such systems to be feasible under certain assumptions. Then we present our algorithmic solution for general systems of more than 2 tasks. Given the increasing and decreasing rates of the tasks, our algorithm returns a subset of the state space such that the system is feasible if and only if the initial state is in this subset. With the knowledge of that subset, a scheduling policy can be computed on the fly as the system runs, with the flexibility to add power-saving, priority-based or fair sub-policies. | |||||
[1] Truong X. Nghiem, Madhur Behl, George J. Pappas and Rahul Mangharam (2011), "Green scheduling: Scheduling of control systems for peak power reduction", In International Green Computing Conference and Workshops (IGCC),. , pp. 1 - 8. IEEE. Orlando, USA. | |||||
BibTeX:
@conference{NghiemEtAl11gss, author = {Truong X. Nghiem and Madhur Behl and George J. Pappas and Rahul Mangharam}, title = {Green scheduling: Scheduling of control systems for peak power reduction}, booktitle = {International Green Computing Conference and Workshops (IGCC),}, publisher = {IEEE}, year = {2011}, pages = {1 - 8}, url = {http://repository.upenn.edu/cgi/viewcontent.cgi?article=1036&context=mlab_papers}, doi = {10.1109/IGCC.2011.6008555} } |
|||||
Abstract: Heating, cooling and air quality control systems within buildings and datacenters operate independently of each other and frequently result in temporally correlated energy demand surges. As peak power prices are 200-400 times that of the nominal rate, this uncoordinated activity is both expensive and operationally inefficient. While several approaches for load shifting and model predictive control have been proposed, we present an alternative approach to fine-grained coordination of energy demand by scheduling energy consuming control systems within a constrained peak power while ensuring custom climate environments are facilitated. Unlike traditional real-time scheduling theory, where the execution time and hence the schedule are a function of the system variables only, control system execution (i.e. when energy is supplied to the system) are a function of the environmental variables and the plant dynamics. To this effect, we propose a geometric interpretation of the system dynamics, where a scheduling policy is represented as a hybrid automaton and the scheduling problem is presented as designing a hybrid automaton. Tasks are constructed by extracting the temporal parameters of the system dynamics. We provide feasibility conditions and a lazy scheduling approach to reduce the peak power for a set of control systems. The proposed model is intuitive, scalable and effective for the large class of systems whose state-time profile can be linearly approximated. |
Workshop and Demo Papers
[15] onathan L Goodall, Madhur Behl, Benjamin Bowes, Brad Campbell, Alex Chen, T Donna Chen, Jeffrey Sadler, Kyle Spencer, Michael Gorman, Shraddha Praharaj, Yawen Shen, Faria Tuz Zahura, Luwei Zeng, "Nuisance Flooding in Coastal Communities: Real-time Modeling and Decision Support to Improve Transportation Infrastructure Resilience"22nd EGU General Assembly, held online 4-8 May, 2020, id.11464 | |||||
BibTeX:
@inproceedings{goodall2020nuisance, title={Nuisance Flooding in Coastal Communities: Real-time Modeling and Decision Support to Improve Transportation Infrastructure Resilience}, author={Goodall, Jonathan L and Behl, Madhur and Bowes, Benjamin and Campbell, Brad and Chen, Alex and Chen, T Donna and Sadler, Jeffrey and Spencer, Kyle and Gorman, Michael and Praharaj, Shraddha and others}, booktitle={EGU General Assembly Conference Abstracts}, pages={11464}, year={2020} } |
|||||
Abstract:Flooding is becoming more common in cities and communities worldwide, causing severe damage and loss of property. Assessing these flood risks and predicting floods rely on a wealth of accurate and trustworthy hydrological information. Recently, increasing attention is being paid to the potential of crowdsourced rainfall networks, with large number of personal weather stations (PWSs) providing high spatial and temporal resolution of rainfall measurements. The highly-localized data collected from these citizen-owned and operated PWSs can effectively bridge the data and information gaps that hamper accurate flood prediction for evidence-based decision-making. However, trust gaps remain in utilizing data from these crowdsourced networks, and methods to measure the trustworthiness of the crowdsourced data in real-time are lacking. In this paper, we present a Reputation System for Crowdsourced Rainfall Networks (RSCRN) to assign trust scores to PWSs based on its agreement, or lack of agreement, with neighboring stations. Using PWS data from the Weather Underground service in the high flood risk region of Norfolk, Virginia, we validate the performance and robustness of the proposed RSCRN. The proposed method converged to a confident trust score for a PWS within the first 10-20 observations from a station and robustly responded to discrepancies in the data due to failure or malicious intent. We benchmark the performance of the proposed method with high-fidelity and trusted hydrological sensor data, which are usually expensive to install and maintain. The results indicate that the trust score derived from the RSCRN can reflect the collective measure of trustworthiness of the PWS, bridging the trust gap for modeling and decision-making in the future. | |||||
[14] Alexander Byron Chen, Jonathan L Goodall, Madhur Behl, "Bridging the Trust Gap in Crowdsourced Hydrological Sensor Networks: Data Trustworthiness of Personal Weather Stations"American Geophysical Union, Fall Meeting 2018 | |||||
BibTeX:
@article{chen2018bridging, title={Bridging the Trust Gap in Crowdsourced Hydrological Sensor Networks: Data Trustworthiness of Personal Weather Stations}, author={Chen, Alexander Byron and Goodall, Jonathan L and Behl, Madhur}, journal={AGUFM}, volume={2018}, pages={H51E--03}, year={2018} } |
|||||
Abstract:Flooding is becoming more common in cities and communities worldwide, causing severe damage and loss of property. Assessing these flood risks and predicting floods rely on a wealth of accurate and trustworthy hydrological information. Recently, increasing attention is being paid to the potential of crowdsourced rainfall networks, with large number of personal weather stations (PWSs) providing high spatial and temporal resolution of rainfall measurements. The highly-localized data collected from these citizen-owned and operated PWSs can effectively bridge the data and information gaps that hamper accurate flood prediction for evidence-based decision-making. However, trust gaps remain in utilizing data from these crowdsourced networks, and methods to measure the trustworthiness of the crowdsourced data in real-time are lacking. In this paper, we present a Reputation System for Crowdsourced Rainfall Networks (RSCRN) to assign trust scores to PWSs based on its agreement, or lack of agreement, with neighboring stations. Using PWS data from the Weather Underground service in the high flood risk region of Norfolk, Virginia, we validate the performance and robustness of the proposed RSCRN. The proposed method converged to a confident trust score for a PWS within the first 10-20 observations from a station and robustly responded to discrepancies in the data due to failure or malicious intent. We benchmark the performance of the proposed method with high-fidelity and trusted hydrological sensor data, which are usually expensive to install and maintain. The results indicate that the trust score derived from the RSCRN can reflect the collective measure of trustworthiness of the PWS, bridging the trust gap for modeling and decision-making in the future. | |||||
[13] Jeffrey Michael Sadler, Jonathan L Goodall, Madhur Behl, Mohamed M Morsy, "Assessing Current and Future Utility of Predictive Active Stormwater Controls for Reducing Flooding in Coastal Cities"American Geophysical Union, Fall Meeting 2018 | |||||
BibTeX:
@article{sadler2018assessing, title={Assessing Current and Future Utility of Predictive Active Stormwater Controls for Reducing Flooding in Coastal Cities}, author={Sadler, Jeffrey Michael and Goodall, Jonathan L and Behl, Madhur and Morsy, Mohamed M}, journal={AGUFM}, volume={2018}, pages={H43J--2608}, year={2018} } |
|||||
Abstract:Low-lying, low-relief coastal cities have seen increased flooding due to climate change and accompanying sea level rise. This problem is expected to worsen. Actively controlling man-made stormwater systems is a potential solution for addressing coastal flooding. Active stormwater control may consist of automated pumps or valves that control water levels and flows in a stormwater system. We present research on the utility of implementing active stormwater controls in an urban setting. Three control scenarios are compared: no control, rules-based control, and model predictive control. The stormwater system and the control scenarios are simulated using the United States Environmental Protection Agency Storm Water Management Model (SWMM5) coupled with a Python library, swmm_mpc, which enables the simulation of predictive control. Since SWMM5 is used as the process model, an evolutionary algorithm is used to find an effective control strategy in the predictive control scenario. High performance computing resources are used to perform the computationally intensive evolutionary algorithm. A simplified model patterned after a coastal city is used to demonstrate the utility of the active controls. The model contains two detention ponds with actively-controlled outlets. In the use case, the control of the outlet of the ponds influenced the flooding of a downstream location. The rules-based approach reduced the amount of flooding by 45% and the model predictive control approach eliminated flooding altogether. Although, the model predictive control approach was most effective at finding a control strategy that reduced flooding it was computationally expensive, taking 36 minutes to run a 16-hour simulation using 16 cores on a high performance computer. In addition to assessing the utility of active stormwater control in a scenario based on current sea-level conditions, we explore how that utility will increase as sea level rises. | |||||
[12] Benjamin Donald Bowes, Jonathan L Goodall, Jeffrey Michael Sadler, Mohamed M Morsy, Madhur Behl, "Toward Forecasting Groundwater Table in Flood Prone Coastal Cities Using Long Short-term Memory and Recurrent Neural Networks",American Geophysical Union, Fall Meeting 2018 | |||||
BibTeX:
@article{bowes2018toward, title={Toward Forecasting Groundwater Table in Flood Prone Coastal Cities Using Long Short-term Memory and Recurrent Neural Networks}, author={Bowes, Benjamin Donald and Goodall, Jonathan L and Sadler, Jeffrey Michael and Morsy, Mohamed M and Behl, Madhur}, journal={AGUFM}, volume={2018}, pages={H21J--1776}, year={2018} } |
|||||
Abstract:Coastal cities face recurrent flooding from storm events and rising seas. A contributing factor to flooding in these low relief areas is the groundwater table, which, already relatively shallow, can quickly rise towards the land surface during storm events. This leads to increased surface runoff entering stormwater drainage systems and a greater probability of flooding. As such, groundwater table forecasts could be an important component of real-time flood forecasting systems, but are generally unavailable. Because traditional physics-based models require extensive amounts of subsurface data that is difficult to obtain, especially in urban environments, this research evaluates two types of machine learning models, Recurrent Neural Networks (RNN) and Long Short-term Memory neural networks (LSTM), for creating groundwater table forecasts. The two types of networks were built with Tensorflow/Keras to forecast the groundwater table response to forecasted storm events and appropriate hyperparameters were tuned using the Hyperas library. Using observed hourly groundwater levels, rainfall, and tide from the City of Norfolk, Virginia, the networks were trained with data from 2010-2016 and tested with data from 2016-2018. Archived forecast rainfall and tide from two large storms in the test period (Hurricane Hermine and Tropical Storm Julia) were then used to evaluate the effect of forecast inputs on model performance. Results indicate that LSTM is slightly more accurate when forecasting the groundwater table than RNN, likely because of its increased ability to preserve and learn from past information. Average root mean squared error and Nash-Sutcliffe efficiency values for an 18hr forecast for the LSTM were 0.06m and 0.89, respectively, and 0.07m and 0.85, respectively, for the RNN. These forecasts could provide valuable information to aid in planning and response to storm events and will become an increasingly important part of effectively modeling and predicting coastal urban flooding as sea level rises. | |||||
[11] Alexander B Chen, Madhur Behl, Jonathan L Goodall, "Reputation system for ensuring data trustworthiness of crowdsourced weather stations",Proceedings of the 5th Conference on Systems for Built Environments; pgs 198-199 | |||||
BibTeX:
@inproceedings{chen2018reputation, title={Reputation system for ensuring data trustworthiness of crowdsourced weather stations}, author={Chen, Alexander B and Behl, Madhur and Goodall, Jonathan L}, booktitle={Proceedings of the 5th Conference on Systems for Built Environments}, pages={198--199}, year={2018} } |
|||||
Abstract:Decision making in utilities, municipal, and energy companies depends on accurate and trustworthy weather information and predictions. Recently, crowdsourced personal weather stations (PWS) are being widely used to provide a higher spatial and temporal resolution of weather measurements. For instance, increasing attention is being paid to the potential of PWS data to improve flash-flood assessment and prediction. However, tools and methods to ensure the trustworthiness of the crowd-sourced data in real-time are largely missing. In this paper, we present a Reputation System for Crowdsourced Rainfall Networks (RSCRN) to assign trust scores to personal weather stations in a region. Using real PWS data from the Weather Underground service in the high flood risk region of Norfolk, Virginia, we validate the performance and robustness of the proposed RSCRN. The proposed method is able to converge to a confident trust score for a PWS within 10-20 observations of installation and can robustly respond to any discrepancies in the data due to failure or malicious intent. We benchmark the performance of the proposed method with high-fidelity and trusted hydrological sensor data, which are usually expensive to install. Collectively, the results indicate that the trust score derived from the RSCRN can reflect the collective measure of trustworthiness to the PWS, ensuring both useful and trustworthy data for modeling and decision-making in the future. | |||||
[10] Achin Jain, Madhur Behl, Rahul Mangharam (2016), "Data Predictive Control for Building Energy Management: Poster Abstract", Proceedings of the 3rd ACM International Conference on Systems for Energy-Efficient Built Environments, Palo Alto, CA, USA. | |||||
BibTeX:
@inproceedings{Jain:2016:DPC:2993422.2996410, author = {Jain, Achin and Behl, Madhur and Mangharam, Rahul}, title = {Data Predictive Control for Building Energy Management: Poster Abstract}, booktitle = {Proceedings of the 3rd ACM International Conference on Systems for Energy-Efficient Built Environments}, series = {BuildSys '16}, year = {2016}, isbn = {978-1-4503-4264-3}, location = {Palo Alto, CA, USA}, pages = {245--246}, numpages = {2}, url = {http://doi.acm.org/10.1145/2993422.2996410}, doi = {10.1145/2993422.2996410}, acmid = {2996410}, publisher = {ACM}, address = {New York, NY, USA}, } |
|||||
Abstract: Decisions on how best to optimize today's energy systems operations are becoming ever so complex and conflicting such that model-based predictive control algorithms must play a key role. However, learning dynamical models of energy consuming systems such as buildings, using grey/white box approaches is very cost and time prohibitive due to its complexity. This paper presents data-driven methods for making control-oriented model for peak power reduction in buildings. Specifically, a data predictive control with regression trees (DPCRT) algorithm, is presented. DPCRT is a finite receding horizon method, using which the building operator can optimally trade off peak power reduction against thermal comfort without having to learn white/grey box models of the systems dynamics. We evaluate the performance of our method using a DoE commercial reference virtual test-bed and show how it can be used for learning predictive models with 90% accuracy, and for achieving 8.6% reduction in peak power and costs. | |||||
[9] Madhur Behl and Rahul Mangharam (2016), "Interactive Analytics for Smart Cities Infrastructures", In First International Workshop on Science of Smart City Operations and Platforms Engineering (SCOPE). [Under Review] | |||||
BibTeX:
@conference{scope16, author = {Madhur Behl and Rahul Mangharam}, title = {Interactive Analytics for Smart Cities Infrastructures}, booktitle = {First International Workshop on Science of Smart City Operations and Platforms Engineering (SCOPE)}, year = {2016} } |
|||||
Abstract: Infrastructures in smart cities are complex systems, more than the sum of their parts, and operate through a multitude of individual and collective decisions. These systems are experiencing a gradual but substantial change in moving away from being non-interactive and manually-controlled sys- tems to utilizing tight integration of both cyber (computation, communications, and control) and physical representations guided by first principles, at all scales and levels. As a result, facility managers for such systems are demanding more control over the system operation. They prefer data-driven insights into performance and usage across their portfolios to make more effective decisions. Such insights help determine, if the system is operating efficiently and enable the facilities manager/system operator to investigate areas for improvement and evaluate upgrades. In this paper we describe one such system, which uses data-driven control-oriented models to build a data intelligence layer for the underlying physical infrastructure. Our system provides interactive analytics for the operator by answering queries and making recommendations about the systems oper- ation. We extend our previous work with using regression trees ensembles for predictive modeling of these large and ’messy’ systems, and show how tree based models can be converted into a knowledge discovery database. We present preliminary results for this system using data from a large office building. | |||||
[8] Madhur Behl Meghan Clark Alexandre Donzé Prabal Dutta Patrick Lazik Mehdi Maasoumy Rahul Mangharam Truong X Nghiem Vasumathi Raman Anthony Rowe Alberto Sangiovanni-Vincentelli Sanjit Seshia Tajana Simunic Rosing Jagannathan Venkatesh Baris Aksanli Alper S Akyurek (2014), "Demo Abstract: Distributed Control of a Swarm of Buildings Connected to Smart Grid", In Proceedings of the 1st ACM Conference on Embedded Systems for Energy-Efficient Buildings. , pp. 172-173. Memphis, USA. | |||||
BibTeX:
@proceedings{BarisAlperBehlEtAl2014, author = {Baris Aksanli, Alper S Akyurek, Madhur Behl, Meghan Clark, Alexandre Donzé, Prabal Dutta, Patrick Lazik, Mehdi Maasoumy, Rahul Mangharam, Truong X Nghiem, Vasumathi Raman, Anthony Rowe, Alberto Sangiovanni-Vincentelli, Sanjit Seshia, Tajana Simunic Rosing, Jagannathan Venkatesh}, title = {Demo Abstract: Distributed Control of a Swarm of Buildings Connected to Smart Grid}, booktitle = {Proceedings of the 1st ACM Conference on Embedded Systems for Energy-Efficient Buildings}, year = {2014}, pages = {172-173}, url = {http://escholarship.org/uc/item/8sd303p9.pdf} } |
|||||
Abstract: The demo illustrates first a method for measuring the effects of individual building management tools on the grid and provide this information to the tools and where warranted, to the building operators to help finding corrective actions. By using a simulation tool, we can forecast any possible instability event before it happens and warn each tool if their actions are likely to affect negatively the grid. | |||||
[7] Madhur Behl, Neel D. Shah, Larry Vadakedathu, Dan Wheeler and Rahul Mangharam (2013), "Demo Abstract: EnergyLab: Building Energy Testbed for Demand-response", In 2013 ACM/IEEE International Conference on Information Processing in Sensor Networks (IPSN). , pp. 303-304. Philadelphia, USA. | |||||
BibTeX:
@conference{BehlShahVadakedathuEtAl2013, author = {Madhur Behl and Neel D. Shah and Larry Vadakedathu and Dan Wheeler and Rahul Mangharam}, title = {Demo Abstract: EnergyLab: Building Energy Testbed for Demand-response}, booktitle = {2013 ACM/IEEE International Conference on Information Processing in Sensor Networks (IPSN)}, year = {2013}, pages = {303--304}, url = {https://www.infona.pl/resource/bwmeta1.element.ieee-art-000006917555}, doi = {10.1109/IPSN.2013.6917555} } |
|||||
Abstract: A building testbed for design and evaluation of energy efficient control and demand response strategies for real buildings is presented. The testbed is a scaled down model of a centralized Heating, Ventilation and Air Conditioning (HVAC) and lighting system. Sensing and control in the tesbed is achieved using the standard Building Automation and Control Network protocol. A MATLAB based front-end can be used to run and observe experiments. | |||||
[6] Truong Nghiem Rahul Mangharam Willy Bernal Madhur Behl (2012), "Demo Abstract: MLE+: Design and Deployment Integration for Energy-Efficient Building Controls", In Proceedings of the Fourth ACM Workshop on Embedded Sensing Systems for Energy-Efficiency in Buildings. , pp. 215-216. Toronto, Canada. [Best Demo Award] | |||||
BibTeX:
@proceedings{mledemo, author = {Willy Bernal, Madhur Behl, Truong Nghiem, Rahul Mangharam}, title = {MLE+: Design and deployment integration for energy-efficient building controls}, booktitle = {Proceedings of the Fourth ACM Workshop on Embedded Sensing Systems for Energy-Efficiency in Buildings}, publisher = {ACM New York, NY, USA ©2012}, year = {2012}, pages = {215-216}, url = {http://dl.acm.org/citation.cfm?id=2422577}, doi = {10.1145/2422531.2422577} } |
|||||
Abstract: While building simulation software tools are excellent at carrying out accurate and realistic building simulations they only provide very basic control methods. On the other hand, control engineers and researchers have explored ad- vanced control strategies for energy-efficient operation of a building, but more often than not, such methods are based on simplified physical models instead. We present MLE+, a tool for energy-efficient building automation design, co- simulation and analysis. The tool leverages the high-fidelity plant simulation capabilities of building modeling software, EnergyPlus, and the scientific computation and design capa- bilities of Matlab/Simulink for controller design. MLE+ fa- cilitates integrated building simulation and controller formu- lation with integrated support for system identification, con- trol design, optimization, simulation analysis and commu- nication between software applications and building equip- ment. In this demo, we will show how MLE+ can be used for systematic design of controllers for energy-efficient building model in EnergyPlus. We will also show the capability of MLE+ to control Heating Ventilation and Air Conditioning (HVAC) devices in buildings through the BACnet protocol. | |||||
[5] Willy Bernal, Madhur Behl, Truong Nghiem and Rahul Mangharam (2012), "MLE+: A Tool for Integrated Design and Deployment of Energy Efficient Building Controls", In ACM SIGBED Review - Special Issue on the Work-in-Progress (WiP) session of the 33rd IEEE Real-Time Systems Symposium (RTSS'12). Vol. Volume 10(2), pp. 34-34. San Juan, Puerto Rico. | |||||
BibTeX:
@proceedings{BernalBehlNghiemEtAl2012, author = {Willy Bernal and Madhur Behl and Truong Nghiem and Rahul Mangharam}, title = {MLE+: A Tool for Integrated Design and Deployment of Energy Efficient Building Controls}, booktitle = {ACM SIGBED Review - Special Issue on the Work-in-Progress (WiP) session of the 33rd IEEE Real-Time Systems Symposium (RTSS'12)}, year = {2012}, volume = {Volume 10}, number = {2}, pages = {34-34}, url = {https://dl.acm.org/purchase.cfm?id=2518172&CFID=565508485&CFTOKEN=79545605}, doi = {10.1145/2518148.2518172} } |
|||||
[4] Madhur Behl, Mansimar Aneja, Harsh Jain and Rahul Mangharam (2011), "EnRoute: An energy router for energy-efficient buildings", In 10th International Conference on Information Processing in Sensor Networks (IPSN), 2011. , pp. 125 - 126. IEEE. Chicago, USA. | |||||
BibTeX:
@conference{BehlAnejaJainEtAl2011, author = {Madhur Behl and Mansimar Aneja and Harsh Jain and Rahul Mangharam}, title = {EnRoute: An energy router for energy-efficient buildings}, booktitle = {10th International Conference on Information Processing in Sensor Networks (IPSN), 2011}, publisher = {IEEE}, year = {2011}, pages = {125 - 126}, url = {http://ieeexplore.ieee.org/xpl/login.jsp?tp=&arnumber=5779077&url=http%3A%2F%2Fieeexplore.ieee.org%2Fxpls%2Fabs_all.jsp%3Farnumber%3D5779077} } |
|||||
Abstract: The US Department of Energy estimates that 73% of electricity usage is consumed by buildings. The Heating, Ventilation and Air Conditioning (HVAC) systems account for almost 50% of the total energy budget. Demand-based pricing for commercial buildings makes it necessary for them to reduce power consumption during peak hours, when electricity is priced at a higher rate. Peaks in electricity demand occur when multiple systems are simultaneously consuming electricity. In this paper, we look at the problem of energy load management for commercial buildings and propose En-Route, a wireless Energy Router platform for control and scheduling of energy delivery to minimize peak electricity consumed by buildings. We have built a scaled model of a building, which generates HVAC dynamics that match an actual building. The Energy Router monitors the temperature, humidity and power consumption in different zones of the building in real-time. It then runs an “energy aware” algorithm that schedules HVAC systems based on the operating conditions of each zone to minimize the peak power consumption of the building. | |||||
[3] Utsav Drolia, Z. Wang, Srinivas Vemuri, Madhur Behl and Rahul Mangharam (2011), "Demo abstract: AutoPlug — An automotive test-bed for ECU testing, validation and verification", In 10th International Conference on Information Processing in Sensor Networks (IPSN),. , pp. 131 - 132. IEEE. Chicago, USA. | |||||
BibTeX:
@conference{DroliaWangVemuriEtAl2011, author = {Utsav Drolia and Z. Wang and Srinivas Vemuri and Madhur Behl and Rahul Mangharam}, title = {Demo abstract: AutoPlug — An automotive test-bed for ECU testing, validation and verification}, booktitle = {10th International Conference on Information Processing in Sensor Networks (IPSN),}, publisher = {IEEE}, year = {2011}, pages = {131 - 132}, url = {http://ieeexplore.ieee.org/xpl/login.jsp?tp=&arnumber=5779080&url=http%3A%2F%2Fieeexplore.ieee.org%2Fxpls%2Fabs_all.jsp%3Farnumber%3D5779080} } |
|||||
Abstract: In 2010, over 20.3 million vehicles were recalled. Software issues related to automotive controls such as to cruise control, anti-lock braking system, traction control and stability control, account for an increasingly large percentage of the overall vehicles recalled. We have developed AutoPlug, an automotive Electronic Controller Unit (ECU) test-bed to diagnose, test, validate and verify controls software. AutoPlug consists of multiple ECUs interconnected by a CAN bus, a race car driving simulator which behaves as the plant model and a vehicle safety and performance monitor in Matlab. As the ECUs drive the simulated vehicle, the physics-based simulation provides feedback to the controllers in terms of acceleration, yaw, friction and vehicle stability. This closed-loop platform is then used to evaluate multiple vehicle control software modules such as traction, stability and cruise control. With this test-bed we are aim to develop ECU software diagnosis and testing to evaluate the effect on the stability and performance of the vehicle. Code updates can be executed via a smart phone so drivers may remotely “patch” their vehicle. We will demonstrate a functioning closed-loop automotive test-bed to show the capabilities and challenges of remote code updates for vehicle recalls management. | |||||
[2] Madhur Behl and Rahul Mangharam (2010), "Pacer Cars: Real-Time Traffic Shockwave Suppression", In In Proceedings of the 32nd IEEE Real-Time Systems Symposium (Work in Progress session - RTSS11-WiP). IEEE. San Diego, USA. | |||||
BibTeX:
@inproceedings{BehlMangharam2010, author = {Madhur Behl and Rahul Mangharam}, title = {Pacer Cars: Real-Time Traffic Shockwave Suppression}, booktitle = {In Proceedings of the 32nd IEEE Real-Time Systems Symposium (Work in Progress session - RTSS11-WiP)}, publisher = {IEEE}, year = {2010}, url = {http://www.seas.upenn.edu/~mbehl/pubs/pacer.pdf} } |
|||||
Abstract: ‘Stop-and-go’ waves or traffic shockwaves is a well analyzed traffic phenomenon and a known cause of traffic congestion. We propose Pacer Cars, a practical, low-cost and infrastructure-less technique to increase highway traffic capacity by alleviating and preventing traffic shockwaves. Pacer Cars are special cars, such that other cars are prohibited from overtaking a Pacer Car. We show that by injecting Pacer Cars into traffic streams on freeways, we can reduce the inflow of vehicles into a traffic shockwave, and prevent traffic congestion from propagating. We formulate traffic shockwave suppression as a control and scheduling problem and present a generic framework that is both practical and effective in suppressing shock waves. We demonstrate how reducing traffic shockwaves can increase traffic throughput without additional infrastructure investment. | |||||
[1] Madhur Behl, Willy Bernal ,Truong Nghiem, Miroslav Pajic and Rahul Mangharam (2010), "From Control to Scheduling: An Elastic Execution Model", In In Proceedings of the 32nd IEEE Real-Time Systems Symposium (Work in Progress session - RTSS11-WiP). | |||||
BibTeX:
@inproceedings{BehlBernalMangharam2010, author = {Madhur Behl, Willy Bernal, Truong Nghiem, Miroslav Pajic and Rahul Mangharam}, title = {From Control to Scheduling: An Elastic Execution Model}, booktitle = {In Proceedings of the 32nd IEEE Real-Time Systems Symposium (Work in Progress session - RTSS11-WiP)}, year = {2010}, url = {http://cse.unl.edu/~rtss2008/WIP2010/8.pdf} } |
|||||
Abstract: We present an elastic execution model for scheduling control systems while maintaining an acceptable level of service. Scheduling allows for coordination, composition and optimization across multiple interacting and non-interacting control systems. Unlike traditional real-time scheduling theory, where the execu- tion time, and hence the schedule, are a function of the system variables only, elastic execution time models found in Cyber- Physical Systems are a function of the physical variables and the dynamics of the system that is being controlled. A task-set is constructed by extracting the temporal parameters of a system from its dynamics. We then provide the conditions for feasibility, optimality and admissibility for a set of tasks. One application will be to implement an ‘energy-router’, which coordinates the electrical demand from multiple control systems to minimize the peak-to-average ratio of energy consumption in buildings. Our work is a step towards developing scheduling theory for cyber- physical systems. |
Patents
Madhur Behl and Rahul Mangharam (2015), "METHODS, SYSTEMS, AND COMPUTER READABLE MEDIA FOR A DATA- DRIVEN DEMAND RESPONSE (DR) RECOMMENDER" United States Provisional Patent Application Serial No. 62/267,817 . Filed December 15, 2015.
[BibTeX] |
BibTeX:
@patent{behl_patent, author = {Madhur Behl and Rahul Mangharam}, title = {METHODS, SYSTEMS, AND COMPUTER READABLE MEDIA FOR A DATA- DRIVEN DEMAND RESPONSE (DR) RECOMMENDER}, year = {2015}, number = {62267817} } |
Technical Reports
[3] Madhur Behl and Rahul Mangharam (2015), "Evaluation of DR-Advisor on the ASHRAE Great Energy Predictor Shootout Challenge". Technical report at: University of Pennsylvania. | |||||
BibTeX:
@techreport{behl_ashrae, author = {Madhur Behl and Rahul Mangharam}, title = {Evaluation of DR-Advisor on the ASHRAE Great Energy Predictor Shootout Challenge}, school = {University of Pennsylvania}, year = {2015}, url = {http://repository.upenn.edu/cgi/viewcontent.cgi?article=1093&context=mlab_papers} } |
|||||
Abstract: This report describes the evaluation of DR-Advisor algorithms on '' The Great Energy Predictor Shootout - The First Building Data Analysis and Prediction Competition'' held in 1993-94 by ASHRAE. | |||||
[2] Madhur Behl, Truong Nghiem, and Rahul Mangharam (2013), "Uncertainty Propagation from Sensing to Modeling and Control in Buildings-Technical Report.". Technical report at: University of Pennsylvania. | |||||
Abstract: One of the biggest challenges in the domain of cyber physical energy systems is in accurately capturing the dy- namics of the underlying physical system. In the context of buildings, the modeling difficulty arises due to the fact that each building is designed and used in a different way and therefore, it has to be uniquely modeled. Furthermore, each building system is a collection of a large number of interconnected subsystems which interact in a complex manner and are subjected to time varying environmental conditions. | |||||
BibTeX:
@techreport{uncer_behl, author = {Madhur Behl and Truong Nghiem, and Rahul Mangharam}, title = {Uncertainty Propagation from Sensing to Modeling and Control in Buildings-Technical Report.}, school = {University of Pennsylvania}, year = {2013} } |
|||||
[1] Madhur Behl (2008), "Mobility Modeling of Swarm Robots". Semester Thesis at: Punjab Engineering College and ETH Zurich. | |||||
BibTeX:
@techreport{behl2008mobility, author = {Madhur Behl}, title = {Mobility Modeling of Swarm Robots}, school = {Punjab Engineering College and ETH Zurich}, year = {2008}, url = {http://www.seas.upenn.edu/~mbehl/pubs/eth.pdf} } |
|||||
Abstract: Autonomous robotics is an area that attracts significant research interest in the robotics knowledge domain. The use of swarm robots for communication and networking purposes is one of their possible applications. The standard algorithms controlling the trajectories of these robot swarms aim at maintaining a given swarm formation in the 2D or even 3D space. Collision avoidance and repulsion are the main operations coming under the control of those algorithms. When looking at these robots from a purely networking point of view one question that becomes relevant is how could this structured movement of the swarm robots be best simulated and to what extent could it be approximated by existing or to- be-developed random group mobility models. The project builds on work on: - Group mobility modeling in 2D and 3D space: constraints are different than the movement within buildings, which has been the main focus of the rather limited 3D mobility modeling work so far - Robot swarm movement control: here basic control algorithms addressing collision avoidance and repulsion in 2D space may have to be extended to also address movement in 3D space. |