The Internet of Wasted Things
Office buildings, schools, stores, hotels, restaurants and other commercial and institutional buildings generate significant amounts of waste. While buildings are becoming increasingly aware of their energy and water usage, the capability to track waste and material use with the same ease has remained beyond the reach of most building facilities managers. Through the proposed work, we will develop a novel approach to waste tracking, and understanding waste behaviors of occupants and help close the loop for waste management for buildings. As organizations create new waste reduction goals, the proposed research methods will lay the foundation for - The Internet of Wasted Things (IoWT)
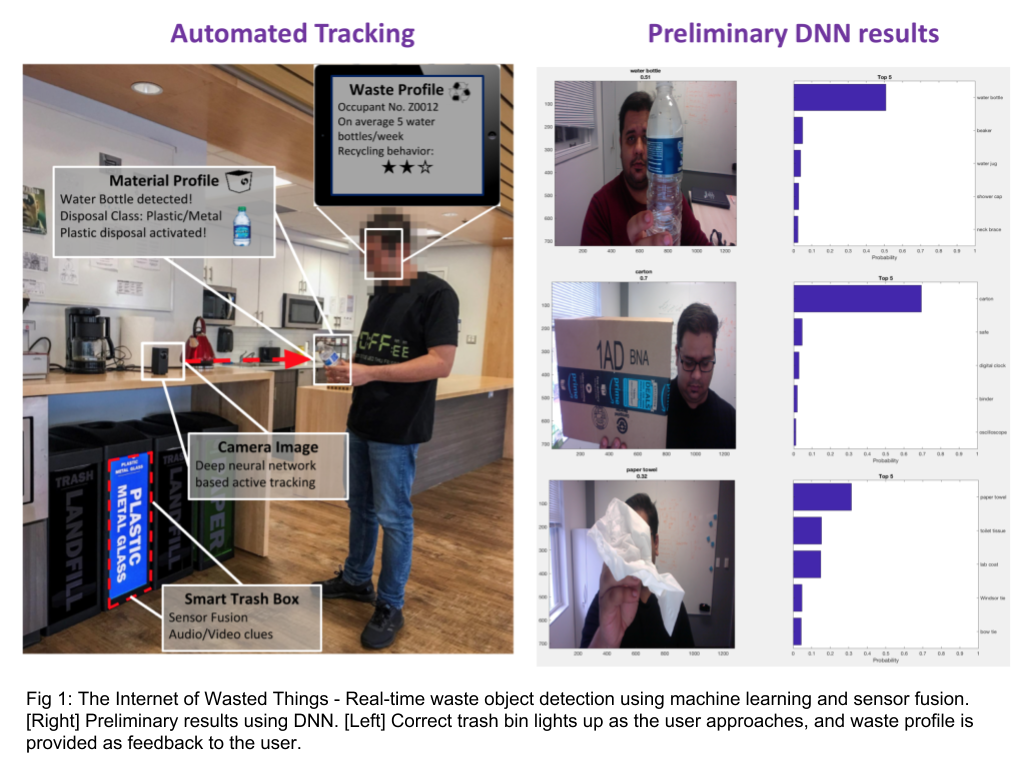
Automated waste tracking & disposal
Tracking can occur at different stages of the waste disposal system. The proposed work intends to categorize the waste type, and quantity at the first point of influx into the waste disposal system - i.e. at the trash can level. We are interested in answering the following research questions:
- What object is being thrown into the trash container(s)?
- Which trash container is the object being thrown into?
- How frequently do occupants dispose away specific trash into an incorrect container?
Using a mix of computer vision methods, combined with sensors fusion techniques will be adequate to answer these questions. Let us begin with the first question regarding the detection of the object being disposed. This is a computer vision problem. Underpinning the computer vision is deep learning. At their simplest, deep neural networks are advanced pattern recognition and allow for machines to draw conclusions from vast datasets. We are building and training a vision based deep neural network (DNN), which can detect and classify everyday waste objects from video data. To this effect we have created a library of ‘disposed objects’ (below) that the DNN will be trained to classify. Our preliminary results using a stock web camera show that it is indeed possible to classify everyday waste objects with significant accuracy, but there is a tremendous room for improvement in these methods. In our experimental setup, we use a camera which faces a trash can, with a field of view, such that it is able to detect and correctly classify the object being thrown into the trash bin. While, computer vision based object detectors have been proven to be successful in the literature, they are not perfect; especially due to real work issues such as varying light levels and occlusions. Therefore, we instead rely on a sensor fusion method. We retrofit the trash can with additional sensors which can provide additional features for detecting the disposed objects. For instance, a weight sensor can help distinguish between an actual fruit being disposed, versus a box with a picture of a fruit being disposed.